2024: the year we give genAI a body
2023 brought us a powerful but intangible general purpose technology. Roboticists have put it to work quickly, and 2024 will make a mockery of 2023. But what does this mean for the world of work?
Okay, wait. Really? Robots with ChatGPT for brains, already?
Yes, really. Here’s a brief rundown on key events in just the last week (each link has at least one video and plenty of supporting material):
On January 3rd, we learned that a large group of researchers collaborating across places like MIT and Google announced Mobile Aloha, a two-arm robotic rig for just over $31,000 in parts. And it’s run on open-source software that learns when humans control it remotely (known as “teleop”). Per the authors, it contains *four* breakthroughs. It: “1. Moves fast. Similar to human walking of 1.42m/s. 2. Stable. Manipulate heavy pots, a vacuum, etc. 3. Whole-body. All dofs teleoperated simultaneously [me: multiple joints can move at the same time]. 4. Untethered. Onboard power and compute.” The key here is that the mobile aloha system could perform complex tasks autonomously with only 50 training examples - even when researchers tried to mess with it by moving objects, closing drawers, and putting chairs in the way.
On January 4th, researchers at Google DeepMind announced SARA-RT: a system that makes transformer-based models more efficient on a robot, and allows users to direct robots through natural language. Basically, they had many robots crawl various facilities. They’d take in image data, use an LLM to describe each scene (e.g., “a table with some fruit and stains on it”), use an LLM to identify potential tasks for the robot (e.g., “put the fruit in the basket”), check which of those tasks the robot’s body could do (e.g., no folding towels because the robot only has one arm), then pick which task to do based on what was least familiar to the entire fleet of robots. That way, each new task contributed maximally to the knowledge of the central system, making all the robots more capable, sooner - with lower compute cost.
On January 6th we were told that with ten hours of training (we’re left to wonder what that means, exactly), Figure’s humanoid robot learned to make k-cup style coffee at normal human speed in the lab, including recovering in creative ways to spontaneous, minor physical mishaps. Truly amazing. Even if the team had been working at this since the CEO’s Dec 26th announcement about the unexpected, transformative power of foundation models for robotics, it’s still amazing. Oh, and this should break a few stereotypes about silicon-valley engineers insisting on bougie, Kyoto-style coffee (ngl, that’s my go-to).
“Oh yeah?” says 1x Robotics on January 8th, when they posted a demo video of their humanoid robot pouring and serving coffee to passersby in a public space. I haven’t seen a demo-off like this since perhaps the Darpa robotics challenge from 2013-2015.
So, what’s going on here? Why does are the LLM and robotics streams are merging at (sometimes literal) lightning speed?
The main reason has to do with what we saw from OpenAI in the late fall: multimodality. Over the course of the year, freely available generative AI went from handling only text to being able to receive images and produce images, and relate these to text as well. So, for instance, you could give ChatGPT a photo and it would tell you what’s in the scene. Here’s a fun example where Ethan Mollick asks the system to identify a very well camouflaged snow leopard in a distant, grainy photo.
Video is “just” a sequence of many images. And cameras and data storage are inexpensive, which means robots take in lots of video. So it’s much easier now for a robot (running an LLM) to immediately identify what’s going on in a scene, identify potential useful actions to take, and direct its control systems to take those actions. Given that these models run in near real-time, this LLM-enabled control loop includes correcting for surprises or errors as the action unfolds. Here MIT Technology review explores how this is being applied in driverless vehicles - which, like our dishwashers, are 🎶robots in disguise🎶.
Before LLMs, parsing what was going on in a scene, identifying potential goals, and taking action that’s responsive to dynamic conditions… these were herculean tasks, subject to incredibly arcane, brittle, and (therefore) specialized software that managed the problem differently. Tabletop physics models were popular. Neurologically-inspired approaches to computation were on the rise (they’re still quite hot). But with LLMs many more roboticists can get in on the action without hyper-specialized, ultra-rare skill.
If you want a deep dive on the robotics*GPT events in 2023, I recommend starting with these three world-class summaries by recognized leaders in this space, and following your nose from there:
Karol Hausman (Alphabet/Deepmind)
Jim Fan (NVidia)
Brett Adcock (Figure)
But, in sum: deployable robotics is just barely springing out of the gate in 2024 with generative AI under the hood, but the progress is rapid, and resources are flooding in.
So what? We’ve been undercounting on AI*Jobs
In addition to rapid, under-the-hood progress in robotics, the last 365 days have seen a lot of analysis and hot air about the implications of generative AI for jobs and work.
In mid-2023, Rob Seamans, an economist at New York University, and colleagues published an analysis of the potential impact of AI like ChatGPT on all known jobs, as registered in a US-government-curated database on work called O*NET. O*NET covers the work activities for 1,016 occupations, breaking these down into 19,265 tasks, like “immunize a patient” or “operate welding equipment.” And I’ve mentioned before that, Daniel Rock, an economist at UPenn’s Wharton School, and colleagues from OpenAI published similar analysis. Both papers aimed to show how much each and every job was “exposed” to the automation that a general-purpose technology like GPT-4 represents. Exposure here means how many of the fine-grained tasks in a job could get a 50 percent productivity boost if the worker used GPT-style technology.
Many folks anchored on to the fact that a very few jobs that were at or very near 100 percent exposed—like blockchain engineer and mathematician. We’re drawn to black-and-white, “total” style change, for one. But also, it struck closer to home for the intelligentsia: it looked pretty clear that it was mostly white-collar workers who were most exposed to a new automating technology. Despite what some immediately claimed, this didn’t mean those jobs were going away—it meant that someone in those jobs would have to change the way they did almost everything, if they used GPT-style technology. That would require almost complete reskilling. But those jobs are rare. The jaw-dropping scope of this reskilling problem becomes apparent when you read the conclusions in these papers that are perhaps less flashy. Try this one on for size: 80 percent of all working adults have jobs that are 10 percent exposed to GPT-style technology. In the US, that’s 108 million people, and around the world, that’s 2.7 billion people who might need to relearn 10 percent of their job.
Now consider this: all that billions-scale exposure and potential change is just from the “mind” side of the human skills ledger. Those two papers only considered tasks that were subject to automation by software that deals with ideas, writing, images, and thought.
But now you know: we’re also making rapid progress on the “muscles” side of things. In fact, the story of 2024/5 is that progress on the minds side is accelerating progress on the muscles side.
Erik Brynjolfsson, Daniel Rock, Tom Mitchell, other colleagues and I are working on a rubric to estimate the exposure of physical tasks to these developing technologies. Preliminary results make it clear that many, many physical tasks in the O*NET database will be exposed to profit generating, robotic automation, especially as LLMs facilitate their development and deployment. Millions more people will be exposed, and in many cases, they’ll be in more physical jobs that would not have been all that affected by intangible, GPT-style automation. So, it turns out that a 10 percent job change for 2.7 billion of us is probably a significant underestimate. More soon as we analyze the data.
There are at least two differences between robots and servers-and-screens AI that should stop us from leaping too quickly in this direction, however.
Robots are not bicycles
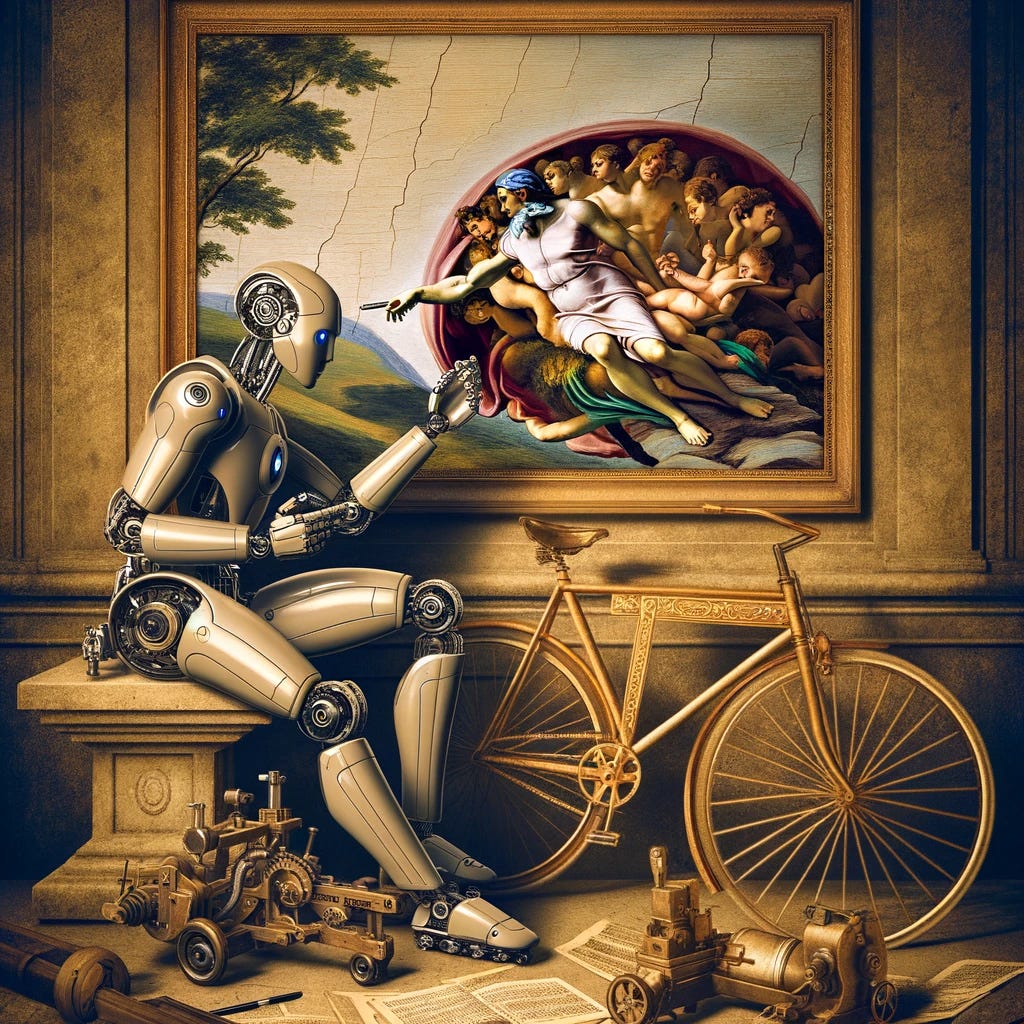
Steve Jobs famously thought of computers as bicycles for the mind. Tools that we build that dramatically amplify our capabilities so when we use them, so we outperform any naturally evolved being on a task (in the case of the bike, energy efficient transport per calorie).
Currently, this is the way generative AI has effects in the world: as force multipliers when a human puts them to use. Think back on those GPT papers above. When the authors are referring to “exposure”, they are talking about tasks where the user picks up some generative AI and uses it to do a task they previously did without the tool. They can often do it faster, better, or both. They might even replace someone who isn’t using the tool, or their organization might not hire more people (thus eliminating potential jobs) by mandating that employees use the tools. But there’s typically no productivity gain unless a human attempts to perform a task with this new “intelligent” tool in their hands. You can gut-check this against your own reality: when was the last time ChatGPT did something without a prompt? Never is the correct answer. Once you prompt it, sure, it does stuff.
Robots are, roughly speaking, the opposite.
A robot is a physical device that can sense its environment, use that data to build a plan for how to act in the physical world, then take that action - and the cycle repeats. You give the robot a set of objectives, define operating parameters (e.g., safety, timing, pace), set it up and let it run. Robotic vacuums are a great example - once they’re set up, they just do the vacuuming. They don’t amplify your personal vacuuming actions. They cut you out of the equation entirely. This is the same with robots in assembly, materials transport, inspection, pick and pack, and yes, even washing dishes. Most robots are like this.
There are notable exceptions: the da Vinci surgical system (the robot I studied for a few years) is notoriously referred to as a “master-slave” system. It takes no direct surgical action without a surgeon pushing or pulling on its controller. We also have powered exoskeletons that give a boost to workers trying to lift or carry something, walk long distances with lots of weight on their back, or for physical rehabilitation. No worker action, no robot action. For wondrous, cutting-edge work in this territory, go look into research on collaborative robotics: software and systems designed explicitly for dynamic physical collaboration on uncertain tasks between humans and robots. Julie Shah’s a recognized leader in this area, and her lab at MIT produces and tests amazing possibilities with corporate partners like Honda.
Let me stress that this is all optional. Robots are not somehow necessarily more autonomous than software. We could have a world where robots primarily amplify human effort or serve as dynamic collaborators. Many power tools now fall in this category. We’ve just built a world where anything we might readily identify as a robot is far more likely to be self-contained, highly autonomous devices.
They offshoot of this difference is that - in most cases - when a task is “exposed” to LLM-enabled robotics, it will be automated completely. Amplifying or collaborative applications are quite rare. So the effect of automation via robot - say in terms of job counts, job composition, and reskilling - will be quite different than those associated with screens-and-servers generative AI. A good rule of thumb is probably: robots mean more replacement, less augmentation. This seems to spell more “all or none” exposure, rather than the “matter of degree” exposure associated with intangible automation.
So there’s your first difference. The second has to do with the fact that generative AI is made of bits, and robots are made of atoms.
Hardware is hard
Before you conclude that the all-or-none exposure insight means LLM-empowered robots will double the amount and rate of job change, take a deep breath and think through what it takes to make something like the robots we see in the news these days.
Or you might instead ask ChatGPT to take a deep breath (as I wrote before, this is one of a bevy of “social programming” techniques for improving output quality) and explain why commercializing robots is slow, compared to software. That’s what I did, and ChatGPT did a fantastic job:
Beyond the reasons above, I’d add two: first, the current and foreseeable cost of a robot’s physical parts and their physical capabilities means that robots are wildly expensive and inefficient way of automating something compared to software. The cost to produce that Mobile Aloha robot above was about $30,000. That’s not including the labor to assemble and test it. And it can only take about one useful action every five to twenty seconds. Sometimes an action takes a minute. And how long would you have to wait for the components to arrive? Oh, you wouldn’t mind if I threw a pandemic or trade war in there to snarl your supply chain a bit, would you? And what if I asked you for a million units?
Second, building and deploying robots means collaborating across many more disciplinary boundaries than in software. Mechanical engineers. Electrical engineers. Embedded systems engineers. Straight-ahead software engineers. I/O psychologists and/or Human Factors folks. Safety and legal professionals. Test engineers. And that’s just inside the traditional design and build team. The research on occupations and professions is crystal clear here (this now-classic paper by Beth Bechky does a great job also summarizing the research): cross-occupational collaboration is harder than intra-occupational collaboration. The more methods, beliefs, values, tools, and practices you have at the table, the harder it is to harmonize them. Software teams are more homogenous in these senses, so the work is easier. Faster.
Improving on any of this takes a massive thicket of interdependent technical and social innovation across numerous disciplinary boundaries. For instance just on the technical side of the ledger, getting a robot to run longer without external power means some combination of advances in areas like battery technology, weight of key materials (in the 2000s we got powdered metal, for example, which reduced the weight of key components while maintaining strength) and power efficiency (here we recently got 3d printed magnets, which can allow for more elegant, energy-sipping actuators - devices that make robots move). All that is slow, hard, and way more expensive per automated action than in software.
Because software is information made animate via controlled energy and we have an internet and distributed computers in place, you can ship and use software at almost zero marginal cost (a term economists use to refer to the cost associated with producing all units of a good beyond the first). Modifying and maintaining it can also be done centrally at relatively low cost, and you can push updates out at zero marginal cost too. It’s all… really easy and fast compared to robotics.
These two factors - robots as replacement and as hardware - mean that it’s not appropriate to directly extrapolate our predictions about the automating impact of generative AI into the robotics space. The implications will take some working out.
But the reality is that generative AI has breathed new life into robotics, and we’re seeing progress on really hard problems to do with the brains side of the robotics ledger. Orienting to an environment. Making sense of it. Planning potential actions. Deciding amongst them. Reacting to surprises. Even shifting goals or trading off amongst them. These hard problems are now easier, for a larger pool of roboticists. And at the same time foundation models are accelerating the basic science underlying many technologies, so the rate of progress on things like power, materials, sensors and even supply chains is likely to take a tick upwards. Making better robot bodies will get easier and easier because of generative AI. So it’s a safe bet that our work is significantly more exposed to robotics in a post-foundation model world than it was beforehand.
The key with this second insight, however, is that it dominates the first one. As much as some top-flight roboticists like to claim, the hard problem in robotics is getting 50,000, 500,000, and then 5,000,000 robots built and shipped to customers that have paid for them. Setting up those supply chains, ensuring six-sigma reliability and safety of those systems… well it’s the equivalent of starting the automotive or home appliance industries all over again. And no matter how inexpensive those robots become, they will never cost $0. Or anywhere close.
I made this point in 2013, and it stands: robots are rounding error in the economy in some senses. They’re really expensive, complex, and difficult to change, compared to software. Both technologies transform and transport things - it’s just that software does it to bits, and robots do it to atoms. Atoms are massive by comparison (sorrynotsorry).
One robot to rule them all
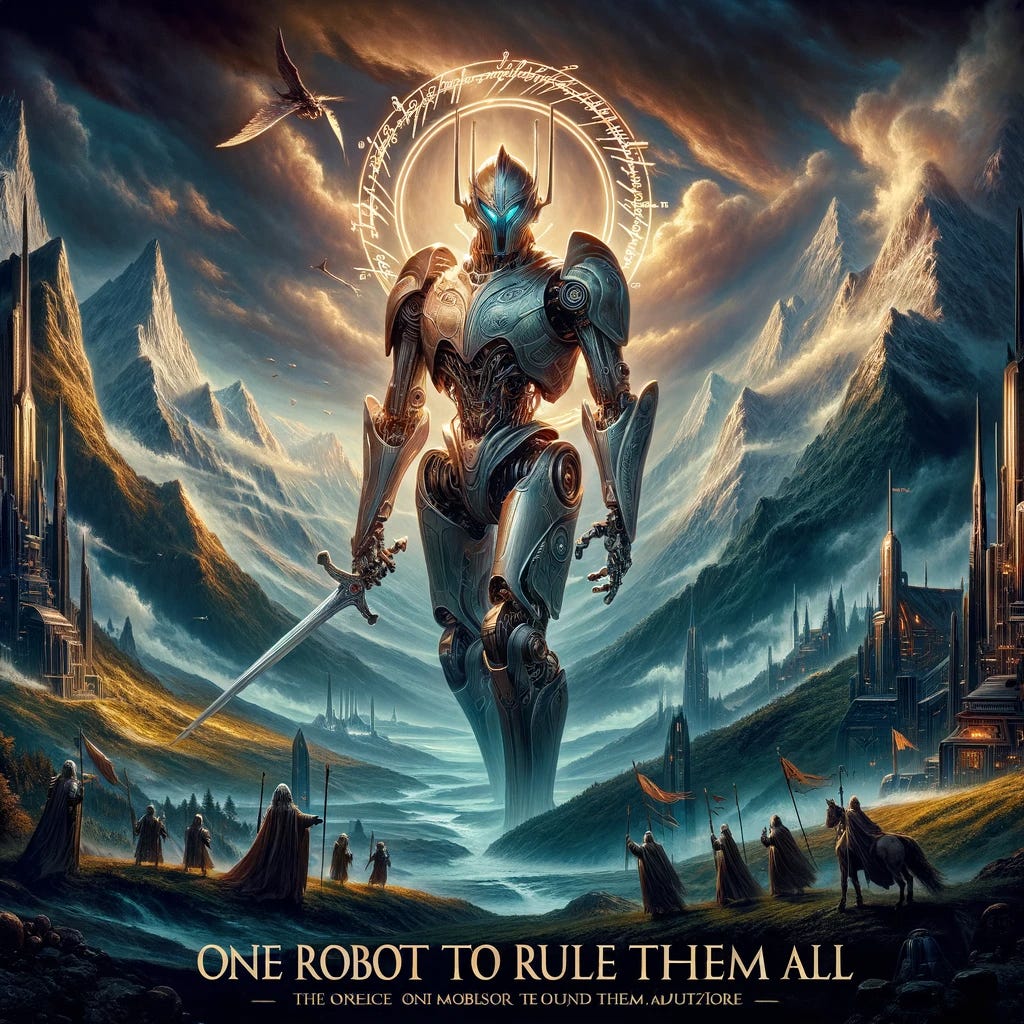
Here’s the potential phase change associated with all this - one that’s worth watching closely: multi-purpose robots.
Until now, most commercially deployed robots have been built to perform one task (e.g., a hulking arm for lifting car chassis around on an automotive production line) or a very tightly bound range of tasks (e.g., a robot that can pull different kinds of cargo through spaces also traversed by humans).
The problem with this 1:1 relationship is that any learning gains associated with that robotic system are hard to port to other robotic chassis or applications. Some of the hardware might be useful in other contexts, but you’d still have to piece together a new rig from a blank sheet of paper. And some of the sensing and control software would probably be useful, but would require extensive tailoring given a new robotic chassis. So the robotics community has sensibly turned to a modular “library” of components - digital and physical - that can be repurposed and recombined without too much hassle. But even all this means that - by and in large - every new robotics project requires a from-scratch design, build, test, order, assemble, maintain work stream that’s quite idiosyncratic to the specific application at hand.
This is part of why investors, roboticists, and some industrialists have been pushing for some time for multi-purpose robots. These are systems that can handle a *very* wide range of tasks with only software changes. The humanoid is the most popular flavor of this kind of system these days. We know very well that the human form can handle a lot of different tasks, so it’s a tested template for a robot that can do the same. A humanoid robot was once a pipe dream, but is undeniably here, now: surf here for my up-to-date list of firms *commercializing* humanoid robots - not just building one that works some of the time.
There’s nothing magical about a bipedal human form for this goal. One of those robots has a wheeled base. Not all of them have five-fingered hands. In fact one of my favorite designs for a multi-purpose robot was CHIMP: Carnegie Mellon’s entry into Darpa’s Robotics Challenge. There’s never been a creature - real or imagined - that quite looked like it, but it could handle a wide range of tasks, some better than a human body could.
But the point is that if we create a standard for a multi-function robotic chassis (or even a few of them), then we could both manufacture and revise it at scale, and most robotic innovation could turn towards improving the software. And software learning gains from one context - how to wrap a gift, sew clothing, assemble electronics, make guacamole, or rivet an aircraft fuselage - could improve these systems’ performance across most other task domains. Then robots could become - as D. Scott Phoenix, the former CEO of Vicarious Robotics repeatedly said - “as ubiquitous and inexpensive as smartphones.”
A lot of diverse parties - entrepreneurs, venture capitalists, government agencies, engineers, scientists - are pushing hard towards this multi-purpose chassis target. Have been for over a decade, actually. But as evidenced by the explosion in my humanoids robot list - and related articles in the tech media - expect there to be a surge of effort in this domain this year and next: everyone recognizes that there’s huge, new value to be unlocked if we can solve this “one robot, one task” problem.
Such consolidation still seems like it’s years away. Say 3-5, at a bare minimum. But as it occurs, our physical work might become exposed to LLMs in ways that beggar the imagination, compared to today.
This doesn’t necessarily mean we’re all out of work. In fact it probably doesn’t mean that, if history and social science offer any predictive value. But it does mean that many more than 2.7 billion of us will have to change significantly more than ten percent of how we do our jobs. Which means that, even more than we might have thought, our short- and mid-term challenges are not job loss, but learning to do our jobs differently.
This is where the multiple roads of automation lead. As far as I can see and the data show, skill development is one of our critical challenges right now, and we’ve got to give it everything we’ve got. Head back to my first Substack post to reorient yourself on that problem if you like, but this is where I’ll focus my posts - perhaps for a while.