Measure twice, spend once
I've learned a fundamental lesson about why we're sacrificing human ability on the altar of productivity: we're not measuring the first, or relating it to the second. We have to fix that - now.
Hi everyone. I’m back. I’ve taken a two month hiatus from my Substack to make more public noise about The Skill Code. I’ve been on 21 podcasts with 16 more on the books. Here’s a recent favorite: Second City’s “Getting to Yes, And” podcast - yes, the improv comedy shop that spawned Tina Fey, Stephen Colbert, Jane Lynch, and Bill Murray. And I have published 24 excerpts, summaries, and OpEds in venues such as the Wall Street Journal, Harvard Business Review, BigThink, Forbes, the Next Big Idea Club, and the Financial Times. Who knows what will follow.
One thing is clear, though: the book has started wonderful conversations, has provoked new thinking, and has instilled a sense of urgency in a wide range of people. Managers and leaders and L&D types came out quickly with reactions. But also parents, uncles, aunts, policymakers, investors and even college students. I’ve been inspired and intrigued by the reviews that these have posted online. Folks are worried about AI. Or complacent. But I keep hearing one thing over and over again - from literally everyone - some version of: “I hadn’t thought about this problem!”
“We’ve started a war between technological productivity and human skill, and skill is losing.” (p139, The Skill Code)
I hear this from all quarters, most notably from those with quite significant power to do something about it: leaders, managers, and human resources professionals in organizations. I’m in a lot more contact with people in these roles now - people with serious experience, a healthy chunk of them running brand-name megafirms making big bets on generative AI in the flow of everyday work. “I have 20,000 Copilot licenses to use,” these people tell me. These people have known for a decade (at least) that we are facing a serious skills gap, and that the way organizations measure workforce readiness is ridiculously out of date, and that AI offers huge, quite uncertain promise as a tool to remedy all that. It’s refreshing to speak with professionals taking these issues so seriously. Yet so far, they have come to me saying the above. Though they might have heard a story or two, and many of them had intuition that something was amiss, they had not considered the possibility that their firms were deploying intelligent technologies in ways that compromised the richest source of skill development they had available: expert-novice collaboration.
Seeing below the surface, through conversation
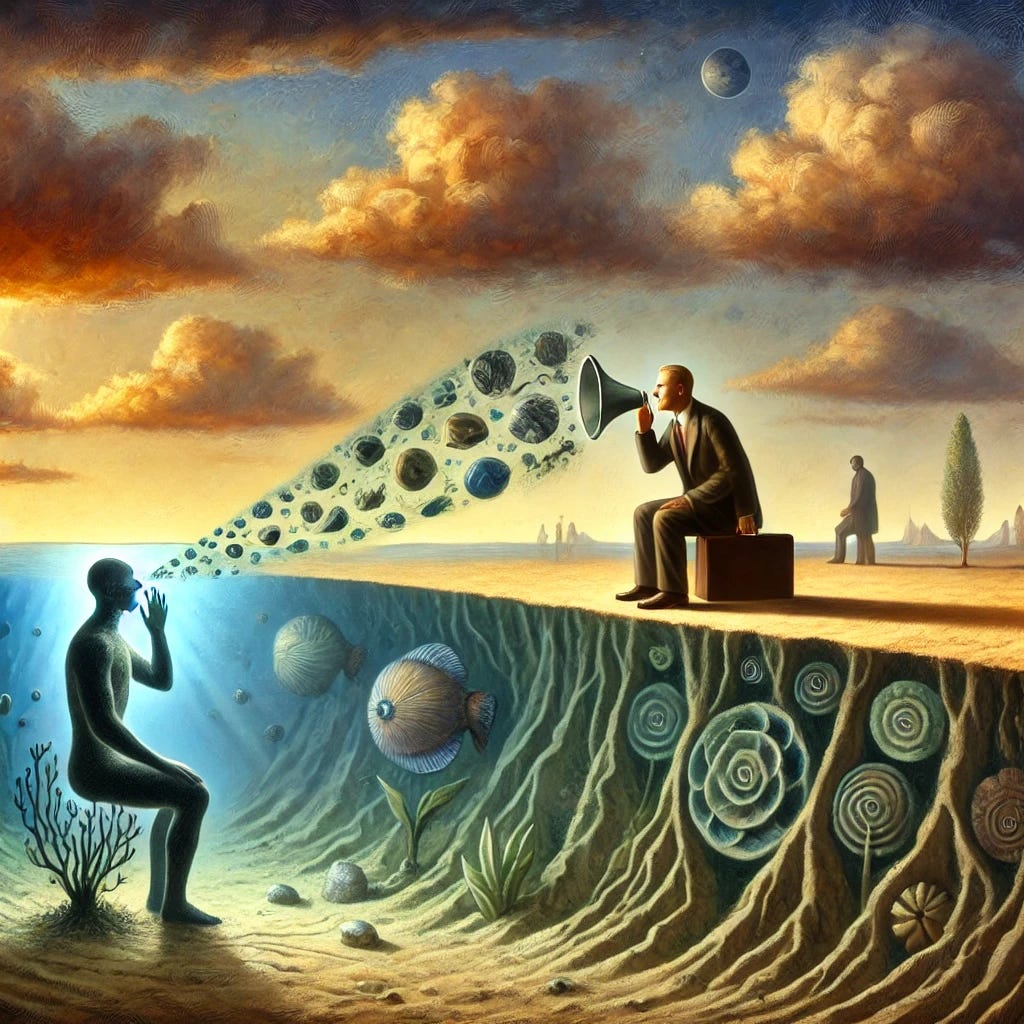
None of this should be surprising. In fact for me it should serve as gratifying confirmation. I had to spend two and a half years in operating rooms in top hospitals, then another three spidering across over 30 other contexts and datasets to see this subtle negative consequence of standard approaches to automation via intelligent technology. Then write a book to clarify my own thinking and bring together the best available data. All my work, primary data, and careful scrutiny should make it perfectly clear the “war” between technologically enhanced productivity and human skill is not exactly obvious.
But hearing all this back from folks made me ask a new question: why?
That is to say: why isn’t this tradeoff between productivity and skill development obvious? I realized I didn’t have a good answer.
We’ve had theories of deskilling from automation since at least the late 80s. But they clearly weren’t top of mind, perhaps in part because they didn’t speak to collaboration across expertise levels. And it turns out the research here is equivocal - in some cases technology leads to deskilling, in other cases it seems to help. Steve Barley wrote a *fantastic* essay on this in the 1980s, basically suggesting that people who found deskilling had looked in places where you’d most expect it to happen.
My stock answer on podcasts - that I still think is not false - has been that no one really “owns” this problem. To begin with, everyone in an organization clearly “owns” productivity. Workers are personally responsible for the quality and pace of their output. Managers and leaders are responsible for the same for the people, functions, and firms they manage. And all parties contribute to and refine ways of tracking progress there. But who owns whether the proverbial saw stays sharp? That’s a maintenance and technical readiness function. Secondary concerns that play out over a longer time horizon that are hard to square with the white-hot concern of more, better, faster, cheaper.
Workforce readiness - a broader set of concerns - is typically owned by human resources, learning & development, and the like. But these folks are often treated (and sometimes consequently treat themselves) as second-class organizational citizens, have limited budget, and spend that budget and their professional attention on issues that revolve around formal training. Worst case they end up focused primarily on compliance training that has little to do with workers’ value-adding abilities, best (typical) case they end up focused on training for the “skills of the workforce to come” or similar.
My book hammers home the main issue there: most of our valuable skill comes through doing our jobs, not formal training. In fact, too much formal training hurts skill development. And we’re definitely doing too much, relative to our investment in the informal process.
None of this is wrong, I’ve realized. It’s just incomplete and misses the fundamental point. There’s something much deeper at work here, something that powerfully explains why the trillion-dollar threat evident in my book isn’t quite on anyone’s radar screen.
You get what you measure, and we’re missing a ruler
tl;dr? We don’t have a measure for the effects of simply doing a task on the skills of the doer, and that stops us from understanding effects of changing that task for the sake of improved productivity.
That’s right, the problem is that we’re missing a ruler.
The research on metrics and evaluation is very clear: until you create a metric to describe something, it doesn’t count, compared to things that are measured. When we invent a low-ish cost way of measuring something, we begin to see it as a legitimate thing to pay attention to, and to shape our decisions around it. Don Kieffer (former VP of operational excellence at Harley Davidson) taught me this when I saw him teaching some executives, and he asked them: what comes first, millimeter-scale manufacturing equipment or the tool that can measure millimeter-scale tolerances in that equipment? The tool, not the equipment. The minute builders, maintainers, leaders, and managers could see that their equipment wasn’t meshing well at that scale, they demanded equipment that could.
I’m talking to a lot of leaders in corporations these days. People who have bought 5, 10, or 20 thousand Copilot or ChatGPT licenses for their workers to use. Some don’t quite know where to deploy them, and are concerned about wasting the opportunity. Some have - a recent survey by UpWork showed 77% of employees feel like their workload has increased because of the technology. Some firms are much more confident because they have a refined sense of where to deploy, thanks to help from my colleagues and friends at Workhelix. And a few of them are, in a sense, past-masters at this already: some high tech firms are over a year and a half into AI deployments for their software engineers. But all of them are hoping for, gathering data on, and measuring one thing: productivity.
A few - a very rare few - have begun to sense that something’s amiss. That pushing so hard for productivity is getting tangible results (at best) but is making it much harder for junior workers to build skill and get ahead. I’m hearing this directly from leaders and managers as I talk with organizations these days. Here’s a recent *superb* essay by Steve Yegge on this topic from inside the belly of the beast (working at AI startup Cody). He flags his essay as “speculative.” He hasn’t read my book, and I doubt he’s aware of my research. If he were, I doubt he’d have put that disclaimer up there.
But none of these firms have data on this sacrifice of human potential on the altar of ai-enabled productivity, or even a clean way of thinking about the problem. The zeroth step, I’ve realized only just recently, is that the only way this issue is going to get noticed or systematically addressed is if the entire world gets a new ruler to measure it.
We’re building that ruler
Last fall, I began working with the fantastic Brandon Lepine (PhD student here at UCSB) to understand how we use generative AI to solve complex problems. If you’re a longer-time reader, you may remember my post that described how we challenged our Master’s students with almost no coding expertise to do data analytics in python and build software useful to a project manager with only genAI as a guide. They all succeeded, in spite of their fear and limited skill. In the background, however, Brandon and I were breaking down their quite complex problems, collecting their chat transcripts, interviewing them, and assessing their knowledge of coding. Why? We wanted to see how they solved problems differently, with genAI in their hands, and what the likely effects of their usage patterns were - on their productivity, sure, but also… their skill. What did they learn, or fail to learn, because they had handed off certain portions of their task to generative AI? Where did they gain in productivity?
We’ve gone a lot further in this direction since. Much more to come on this in a subsequent post, but the short story here is that we are working with some very exciting partners (maybe, next time, I’ll have their clearance to share) to develop a method for examining work that will allow fine-grained predictions about productivity and skill at the same time. That’s important - essential, really - for two reasons. The first is solely focused on productivity. The amazing fact is that while firms have some course measures of productivity changes as a result of genAI deployment, most don’t. They’re just handing out licenses, doing a bit of training, some experimentation, and mostly… hoping. Honestly. And for those that did have good measurement practices and good quality data, they don’t really know why they’re seeing the results they’re getting. They can make coarse distinctions. Useful, but not precise enough for great decision making. Our approach offers dramatic resolution improvement there.
But the more interesting, likely more valuable reason is that right now we think of productivity as what you can get as you automate and redesign work to suit - but not that it might come at the expense of human ability. Again, this shouldn’t be a big surprise, because the core message of my book is a surprise. Leaders, managers, HR and L&D professionals, and even workers usually agree hear that these things are interrelated, and they likewise agree with the finding that says we typically trade away skill development for productivity. But out there, on the ground, this isn’t a ready intuition. That means that as we shoot for increased productivity through genAI-driven automation, many of us will miss situations where insisting on automation patterns that *improve* human ability might actually help you get even more productivity than if you were measuring and intervening on productivity alone. We don’t have science that explains when and where these “both/and” opportunities will emerge, but my and others’ research makes it clear such outcomes are systematically achievable.
Juho Kim - a truly brilliant HCI researcher and collaborator of mine over the last four years - got involved in this effort recently, and he recently framed all this in terms many data-driven managers would relate to: joint optimization.
I talked a lot in the last two chapters of my book about the positive role technology can - and in my view, must - play in a skills future that is brighter than we ever could have achieved before. But if you read that closely, you’d see: I explored examples of how we could use technology to enable better, richer work and human collaboration - not how we could use AI to even *measure* the problem in the first place, so that people would be motivated to address it.
Now, we’re clear: until we have a ruler that measures (and therefore can predict) the interdependent effects of generative AI deployment on productivity and human ability, we’ll get dragged towards the former at the expense of the latter. This of course goes for many other kinds of automation via many other kinds of intelligent technologies, so the returns to this kind of new metric should unfold at the same scale as the problem.
As I said, stay tuned - Brandon, Juho, our collaborators and I will have results to share soon. And if you think your organization, division, or functional group is implementing generative AI in a way that subtly (or not so subtly) sacrifices human ability on the altar of potential productivity - drop me a line. You might just be able to join in on our early efforts to build just the ruler you need to find out, and to bend the arc of implementation towards a win in both categories.
Really helpful and important post Matt