Don't Let AI Dumb You Down
Using AI can improve results... and deskill us. Here's how to boost skill instead.
“Oh my god I’m so lazy now? I mean I just give it an article and say ‘read this and do my assignment.’” - Top undergrad student of mine, chatting with peers in class
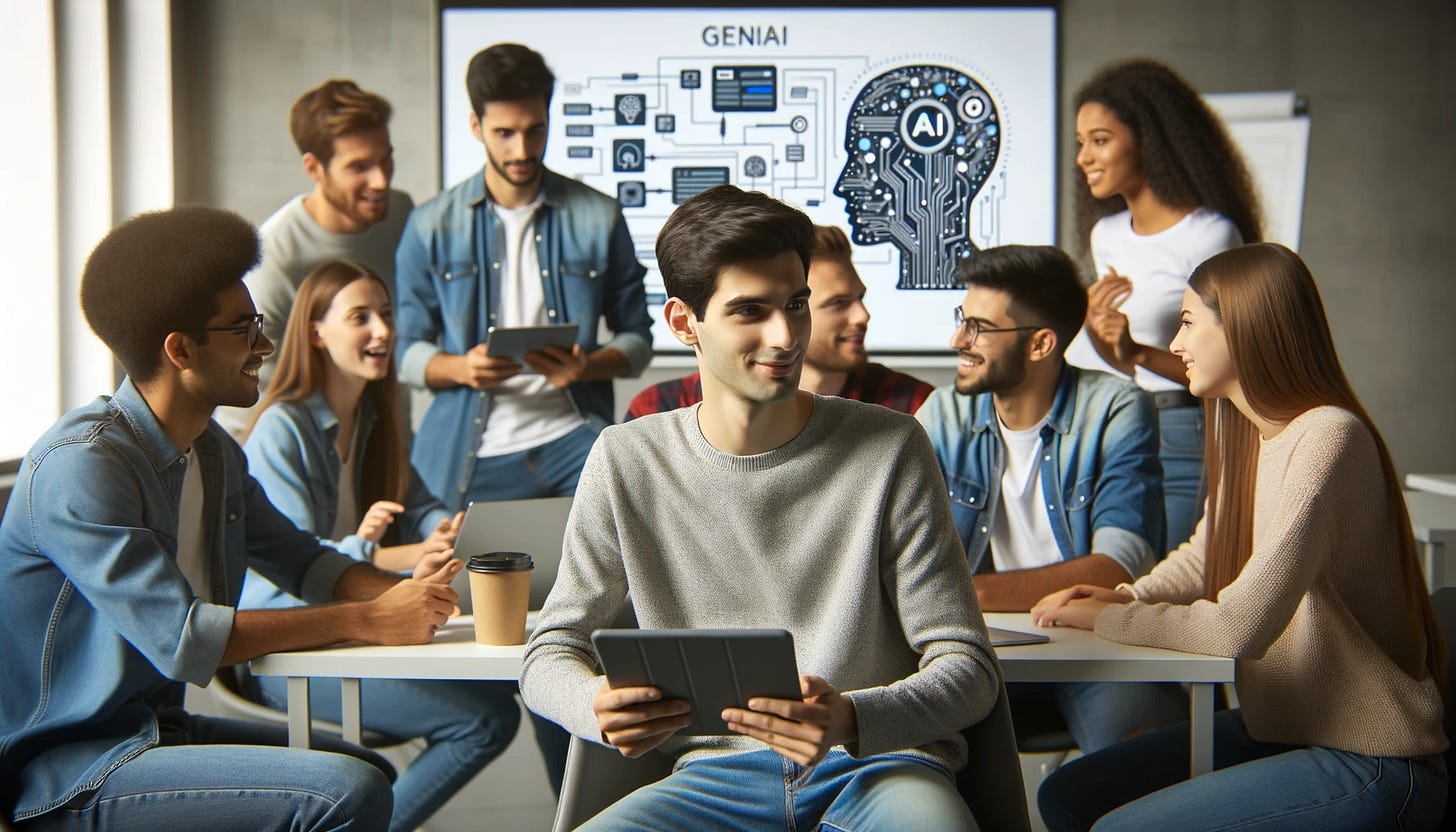
Hundreds of millions of us use genAI.
One of our most common tasks? Writing resumes, cover letters and applications to go after jobs. Some of us are even using it to respond to live questions in remote interviews. And we all know many students use it for homework - writing papers, essays, research reports and so on. Some teachers are grading that submitted work with genAI, by the way, and building tools to help others do the same. Other tasks are more mundane - we use it to write an email, to think through a recipe, to do travel planning, and so on. And far beyond the mundane, very high skill workers are a year or more ahead of us, applying specialized, often pre-ChatGPT genAI in their tasks. Software developers have had a genAI assist at least since GitHub copilot was introduced in 2021. Professional writers, lawyers, and consultants have been using these tools to create, edit, and summarize text. And scientists across numerous disciplines have been using it to find new research questions and directions, design new instruments, collect data, and write up papers.
And it’s working: the immediate results are striking. (There’s a hidden cost, too - I’ll get to that in the next section).
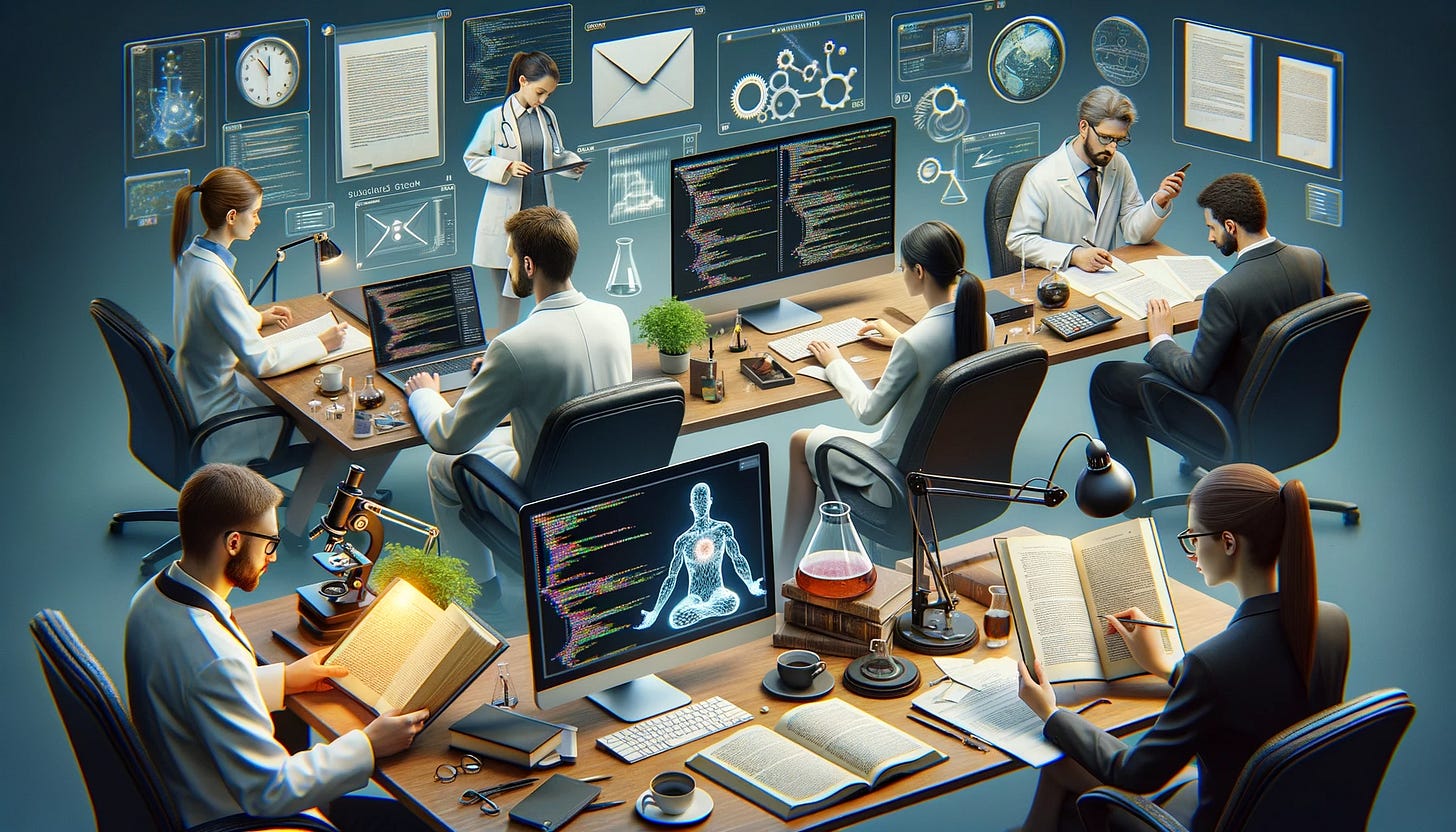
Some waste effort and rework aside, resumes are getting us jobs. Homework is getting better grades. Emails are more persuasive and clear. And not for specious reasons. The materials are better than they would be otherwise. I’ve told my students that I’m expecting better writing from them, now that these tools are here, and they’re delivering. And we can do more of all of this, faster. On the professional side, Software developers are writing better code, more than twice as fast - while feeling more satisfied and focused. Writers get their work done 40% faster and 18% better. Consultants are getting improved results, too. As for scientists? For them it’s letting them “revolutionize the entire data science pipeline”, propose new protein structures, and optimize magnetic containment of superheated plasma for next-gen fusion power.
Finally, society-level productivity gains from genAI should be significant, because we use it a lot: in the last month, chat.openai.com and bing.com (another instance of GPT-4 hosted by Microsoft) had 2.7 billion visits combined. Reuter’s recent poll indicates that 28% of working Americans - that’s just over 4.5 million people - use the technology “regularly” at work, despite the fact that 10% of employers ban its use outright. And while we don’t have good data on this yet, it seems clear that productivity gains will emerge because folks understand that this is erratic technology. Most of us get better results with these systems because we know they “hallucinate” regularly, that you need to check their work, and that new versions, plugins, and competitors are arriving regularly. Beyond current stats, all of this activity - from use across skill levels to sheer usage volume - is increasing and expanding. We’re still finding out ways to put this general purpose technology to use.
So, to sum up: hundreds of millions of us are regularly using generative AI and getting more, better results sooner. Great, right?
Be careful what you wish for…
It can be. Let me stress this: it will sometimes be wonderful to boost quality, volume, and ease of work for the 2.6 billion working adults who can apply this technology in ten percent of their jobs. In the grand sweep of history, this can give us the productivity boost we’ve been missing, creating new value for everyone.
But there is always a tradeoff, and here, it’s about deskilling.
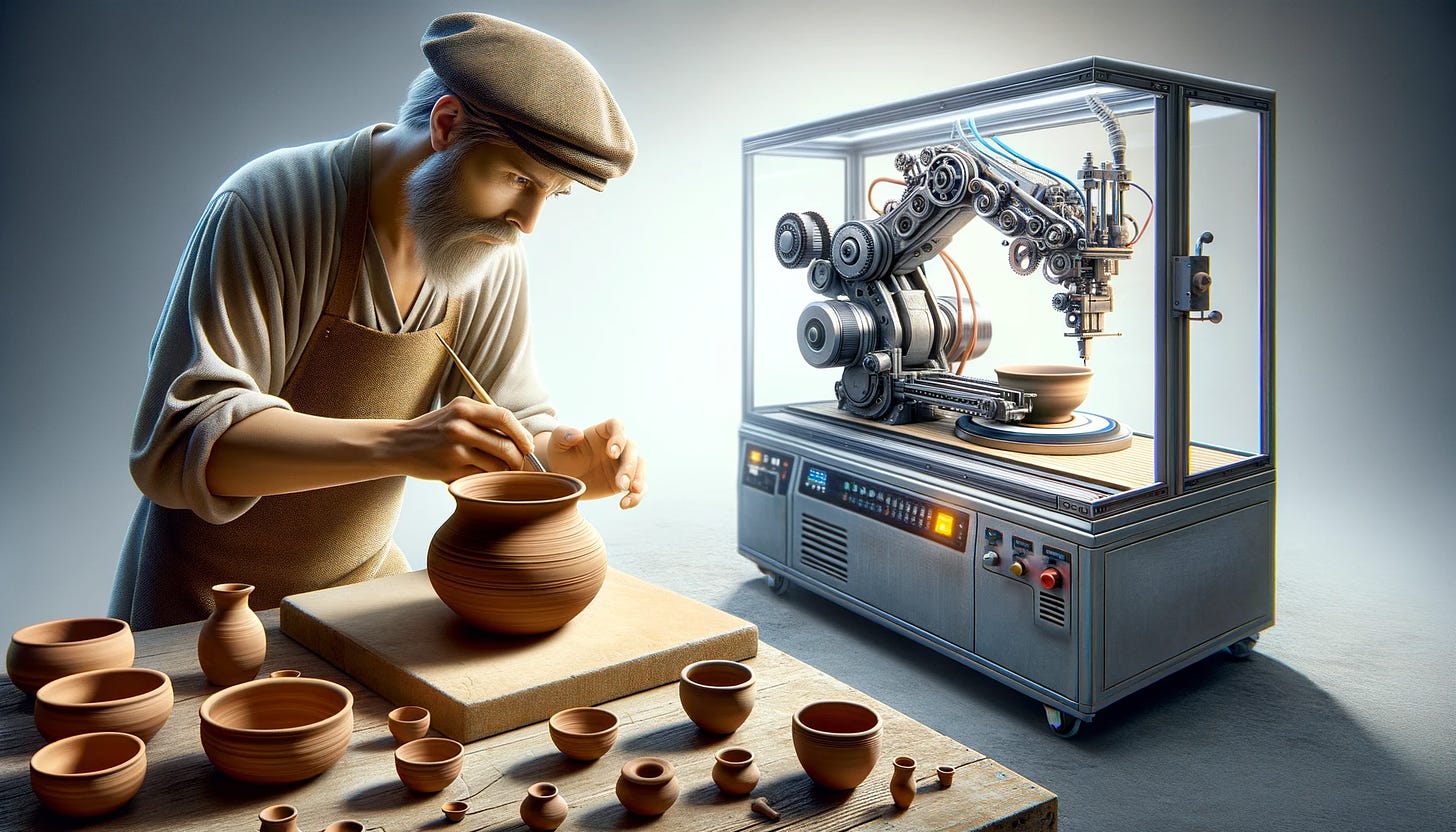
Since the 18th century, we’ve understood that we sometimes lose skill - and the advantages that flow from it - when new automating technologies arrive. Part of the reason for this is routinization: to take get the best results from a new technology, we simplify the work involved. Invented a conveyor system for parcels? Good, now the human job of moving parcels doesn’t require walking around or dealing with other people. Invented a calculator, or an electronic spreadsheet? Great, now the human just enters the data and hits enter. No arithmetic or cross-checking required. Throughput and quality go up, but that new work system demands less of the humans involved.
To illustrate: here’s a quick vignette from Jax (not his real name), a 25-year automation veteran in a leading AI-enabled robotics startup in silicon valley - recorded during my team’s three-year, nationwide study of advanced automation in warehousing:
“So let’s say an injection molding system requires three operators to run at its manual capacity. When they shift it to an automated status, now it's two operators. In that three person configuration, there was probably actually a fair amount of skilled labor that was involved. The local supervisor knows damn well that that job before took a fair amount of savvy - sort of like, ‘come on Bessie now, let's get this part [produced].’ And now it is just push button.”
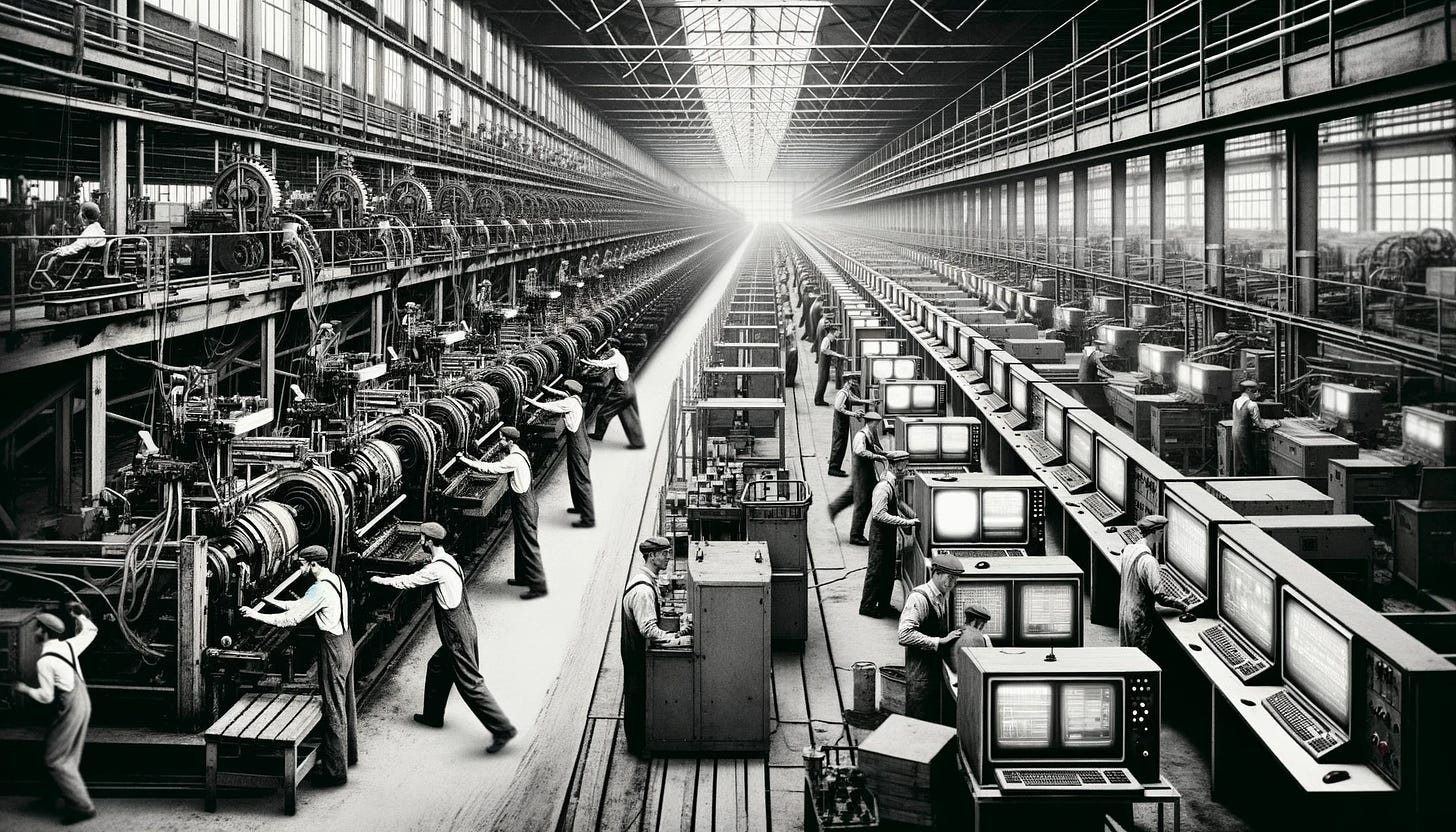
Working with the new technology makes these workers more productive - higher quality parts, faster. And it’s less demanding - not as dangerous, not as stressful, or difficult.
And it’s deskilling. Pouring plastic beads into a hopper, pushing buttons, and inspecting parts takes less ability, so the longer a worker does this new job, the less capable they become. They literally lose skill. New workers in this job simply don’t get a chance to build it. And often, deskilling drags down job quality with it, including pay and dignity. This is the well-accepted finding going back to the 18th century, stretching from Smith to Marx, Braverman to Burawoy, Fernandez to Vallas, right up to today.
Many will counter this argument by saying that rational employers will reallocate humans to tasks that do require (and therefore develop) human judgment and skill, because that’s where human capability is most valuable. And so the net effect of automation is good for the average individual, organization, and certainly society.
This is misleading in two ways in general, and one way that’s unique to genAI.
First, you can’t move everyone to a new, better job that requires and develops valuable skill. A good number of humans have to do the new tasks in the midst of the newly automated process. You leave two operators on the injection molding machine. Instead of two paralegals reviewing contracts, you have one with an AI assist. Instead of two surgeons to do an operation, you’ve got one - and according to many of them across 18 of the top teaching hospitals in the US, compared to open surgery, robotics is like “bumper bowling” - a little too easy for comfort. Without extensive cross training, self-teaching, or other managerial attention, those folks are at risk when it comes to skill.
Second, managers aren’t rational. They’re not all knowing and all seeing. Multiple streams of research show they generally see things the way they saw them yesterday, and are therefore predictably blind to new opportunities, existential external threats, and internal friction in their own firms. Bob Sutton, one of my intellectual heroes, has a new book coming out about this last topic with Huggy Rao. It’s stunning how mucked up organizations can get while persisting for decades. Beyond this, managers and other professionals are satisfied with small, quick wins with new technology. In 1991 we got an absolutely superb (and woefully overlooked) piece of research on this from Marcie Tyre and Wanda Orlikowski. They found that after just 12% of the expected implementation time, most organizations simply stopped changing because they’d gotten a significant productivity boost. So they left most of what they could have gotten out of the tech on the table, and certainly didn’t look into collateral effects like deskilling.
The third point is that genAI is mostly getting rolled out by individuals, and managers may not notice. Over the last 18 months, we’ve tossed a free, internet-accessible version of the most powerful general purpose technology in a generation into the hands of billions of working adults. Some of us ignored it. Some of us wasted our time with it. But many millions of us have used it to do more, better than we could before, because producing content takes less skill and time than it used to. And we don’t need to tell anyone that we’re using genAI, nor is there a way to tell if we are: this is what Ethan Mollick calls the “secret cyborg” problem. The problem for reallocating labor to avoid deskilling in this case is that managers can’t optimize what they can’t detect, so it will take them quite some time to realize why some of their employees, contractors, colleagues, or even bosses are producing a lot more quality work, faster. They may never recognize it, and so leave valuable talent cranking away on increasingly rote tasks when they could be allocating it to more complicated work.
The stereotypical economist (I don’t know many of them, to be honest - the ones I work with are very well attuned to these dynamics) might argue that organizations like this are doomed, and in the long run they’ll be replaced by more efficient ones that allocate workers in skill-enhancing ways. But they know from one of their own brightest stars that this won’t much matter for you, your colleagues, your kids, or your friends:
‘The long run is a misleading guide to current affairs. In the long run we are all dead,’
- John Maynard Keynes, A Tract on Monetary Reform (1923!).
The upshot of all this is that unless we take proactive steps to avoid it, we’re all at serious risk of becoming like the push-button operators of that automated injection molding machine. More throughput, better quality, sure - but stuck in that situation in ways that subtly degrade our skill the longer we remain. And because we’re all driving our own boats this time - management-directed efforts to integrate the technology are quite rare and very small scale - we’re also on our own when it comes to protecting our journey to more valuable and satisfying skill.
SOS - Save Our Skills!
What can we do to avoid deskilling ourselves as we use genAI?
For today, I’m going to focus on the immediate, and the personal. Thankfully, there’s a simple step you can take right now that will drag you away from the slippery slope of deskilling. The more you do this, the better off you’ll be.
This involves using the “custom instructions” capability that OpenAI has rolled out to all users - free and paid. Basically you’re going to tell ChatGPT to nudge you towards more skill in every interaction.
Here’s how to do it:
Log in to chat.openai.com, and go down to the bottom left hand side of the screen to click on your settings. You’ll see the custom instructions option right there:
Click on that, and you’ll see a window like this:
This is a place where you can use natural language to program ChatGPT on how to program you. That’s right. You can tell it what your values, preferences, beliefs, or goals are in the top box, and give ChatGPT specific tactics in the second box to make your actions move your work closer to your values.
It just so happens that - if you buy the article above - you value your lifelong journey to skill, and you want to enhance that while supercharging your work with generative AI. So tell it that!
Starting about four months ago, here’s what’s I put in my custom instruction boxes - both are a work in progress, so have changed slightly as I’ve gone along:
My “about me” box:
“I'm looking to learn and build skills. I need a healthy amount of challenge (working near the edge of my skill), complexity (engaging with the broader context for my task to discover new skills I wasn't aware of), and connection (warm bonds of trust and respect).”
My “How would you like ChatGPT to respond” box:
“Help keep me challenged: ask me if I can confidently do what I'm asking you to do. If I say I can't, suggest a portion of the work I could do on my own instead of asking you to do it. If I say I can do it, inform me of more comprehensive or elegant solutions, and ask if I want to learn how to produce them.
Help me engage with complexity: give me a brief overview of skills, work, roles, and other contextual information related to my task that I might not get if I just got the job done. Also remind me at the end of our task to reflect on it later, to cement my learning.
Help me build human connection: right off the bat, suggest ways I might build bonds of trust and respect with others interested in what I'm doing. Either peers, people who know less than me, or people who know more than me. Be very creative here, and remind me of the importance of this at the end of our work.
Also, offer to debrief complex interactions just before they're concluded. If I agree, begin by offering what each of us did that was particularly helpful, then offer what you think each of us could have done differently to make things even more effective. Then ask me for my reactions to your assessment, as well as my own assessment.”
[I have more text in this box, by the way, I’ll leave that for another post].
Practically, this means I have to read a few more lines of text after my interactions with ChatGPT. Then I have to make a couple extra choices: do I want to learn more, or do I just want my results? Do I want to understand how the injection molding machine works, or how inventory control is done, or more about the different materials the machine works with, or about labor process… or do I just want to produce my widgets? It’s my choice, so while it can be mildly annoying at times, I feel like I’m in control of my skills journey.
And the debrief component has turned out to be very helpful. That should come as no surprise: we have a mountain of research that shows debriefing aids individual, pair, and team-based learning. Why not debrief with your computational prosthesis? Here I’ve learned how to optimize prompts, avoid hallucinations, and to skip steps altogether. Those are all “meta” skills - skills required to build more skills as I use the system.
I’ve learned new skills in coding, data science, cooking, experience design, musicology, and even natural language processing (a form of AI) this way, and I learn one or two things like this every couple of weeks. My learning curve is speeding up, not slowing down. And I take a moment about once every couple of weeks to tweak the contents of these boxes in search of better results. Have you tried anything like this? Drop your experiments in the comments!
Everyone for themselves is no way to win
I’ve given you a right-here, right-now tactic that you can use to protect your current skills and nudge yourself to more and better ones. But we need to do far better than this if humanity is going to survive the tsunami of productivity that’s breaking on our shores. We need to organize and invest here to realize the skills boon that we need to contend with all the change we’re creating.
Eventually I’ll offer some tactics and principles here, but I believe the atomic unit of progress sits just above. Managers, how could you design your organizations to nudge your people towards skills they desire, just by using genAI? Alternatively, what if you asked genAI application vendors to design their tech to do the same? And technologists, try treating this as the huge market opportunity that it is: how could you design your systems to nudge us all towards the skills we’d like to build, instead of simply being the operators of your ever-more sophisticated and capable models?
We need all hands on deck to avoid a massive wave of deskilling. For now, I suggest you get your hands on your custom instruction oars and start to pull.
Great insight - @mattbeane is worth a follow