GenAI means it's harder to trust expertise
When genAI can give us high quality ideas, assessments, and solutions on demand, it's harder to tell if we're dealing with "real" skill. The way forward is simple, not easy.
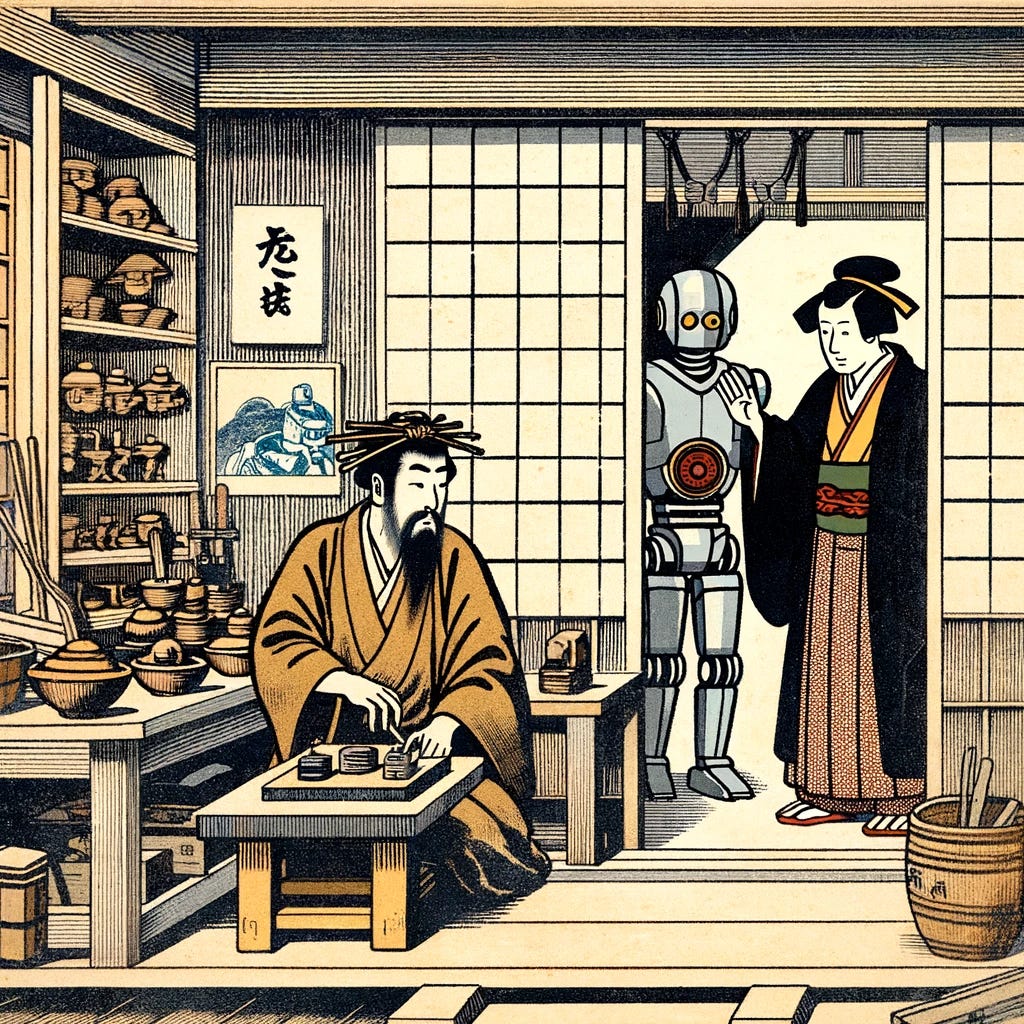
With genAI - ChatGPT, Bing, or Google’s hot-off-the-presses Gemini - all of us have new ways to do our work faster. Better. You probably know this firsthand and from people you know. A letter. A job application. Some homework. All done in minutes, not hours. And if you doubt the anecdotal, you can turn to a growing crop of rigorous studies (here’s my favorite recent example, known as the “Jagged Frontier” paper) showing these effects - improved productivity with essentially zero training. The raw interface is intuitive, thanks to over two decades of global smartphone use: we just chat with these technologies via text, upload documents and images, and we get back high quality text, code, and images in return. It’s really quite something: even after hundreds of hours of use, the results still inspire awe for me.
So in a very real way, we’re more skilled. With genAI in our hands, we have the ability to get higher quality results more reliably and quickly, compared to those without it. What else is expertise, other than the practical ability to solve a problem?
A lot, it turns out. And the “other” part of expertise is under threat.
Expertise - like most human phenomena - is social, not just practical. It boils down to trust: our belief that someone claiming to be an expert is credible and capable. Callen Anthony and I discuss these two aspects of expertise in our recent paper. The problem we found in our data is that senior members of an occupation struggle with building skill with new technologies, because appearing as if they are novices is problematic. People - both the public and junior members of the occupation - need to believe that someone knows what’s going on and how to handle it.
It’s not good enough for us to improve our ability to resolve problems, and for others to do the same. We need to find a way to trust that people have skill before we see it in action. Otherwise experts won’t get the opportunity to work in the first place, and the system breaks down.
Expertise is in “genAI check”
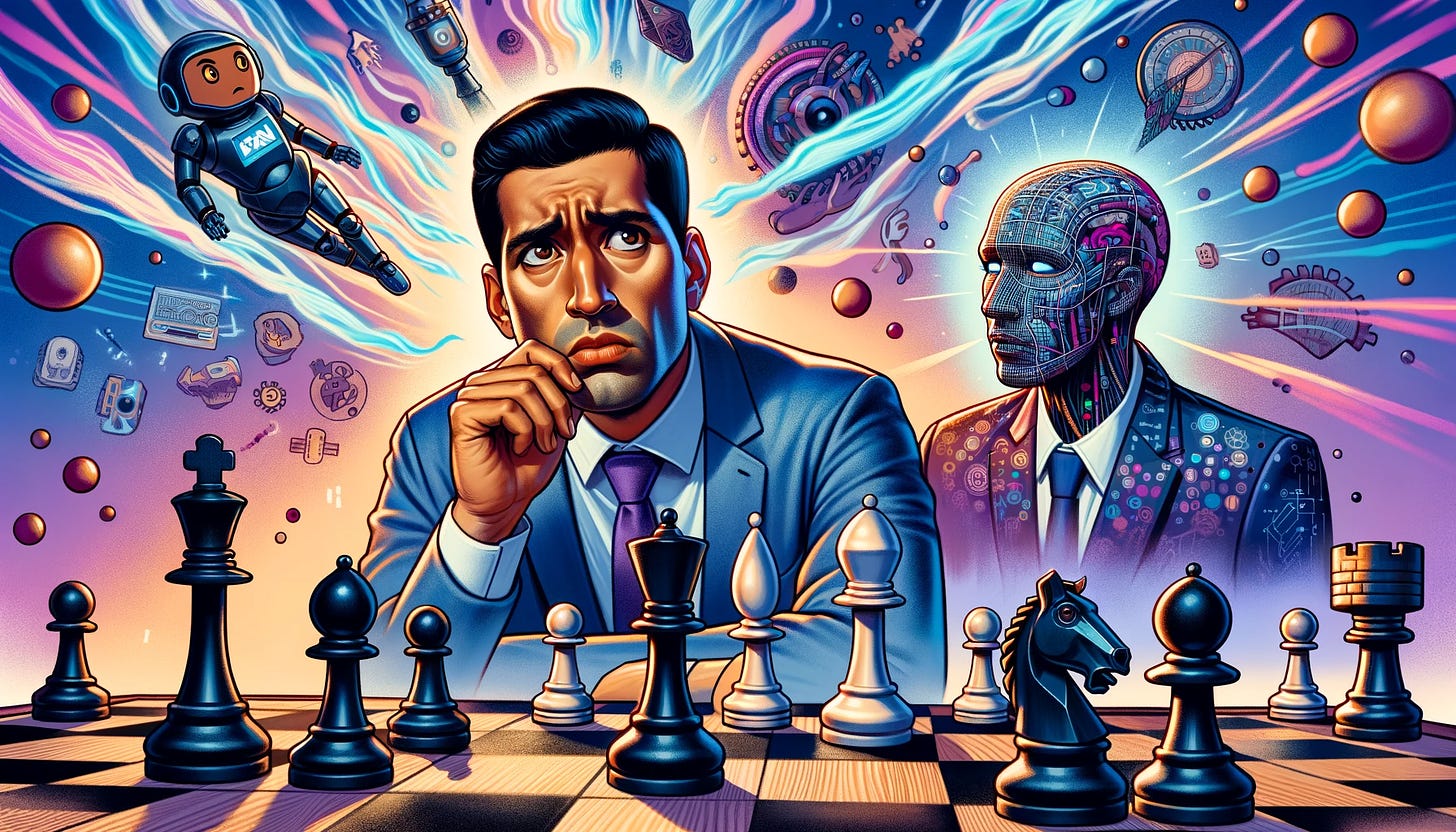
I’ve mentioned before that using genAI threatens skill in one subtle way. You can limit fruitful struggle, creative detours, and interaction with others, which in turn can limit your skills growth. We don’t have to settle for that. In that post I give some tactical guidance to not only avoid the brAIn drAIn, but to get the opposite result - better skills than you could get without AI.
Here I’m focused on the credibility threat to expertise, and will land in a comparable place. Yes, there’s a way to go from being in genAI check on your skill to the opposite: using genAI itself to present our expertise in a new way that enhances people’s trust.
But let’s start with genAI check, and begin on the practical side, closest to “actual” skill.
When you’re hiring someone… when you collaborate… when you join a team… these are all moments when you have to decide: are they good enough?
Even if you’re hiring a contractor to solve a specific, apparently obvious problem, you’re coming to them because you’re not sure about the best way to interpret and act in the situation. You know your gas oven intermittently makes a poof noise, seems to go out and then sounds like it restarts itself, but you don’t know that for sure. Or perhaps you have some extra cash and are looking to invest it for the long haul. You hear that an index fund is smart for a nonprofessional investor, but you don’t know if that’s true in your case, and if so, which one to choose.
All you know is something’s wrong, or there’s an opportunity in front of you.
Research on expertise makes this clear: when you need to trust someone’s expertise, you’re concerned with more than whether they can handle a particular challenging task well. You’re not sure what the task is! You need to trust them to assess a complex situation well, choose an effective method to address it, and then reliably execute on that method. And this is a far bigger deal if you’re going to have multiple interactions, or the problem is really ambiguous or dynamic. You’ll need them to repeat this process for the same problem multiple times, for new problems that are different. And as long as we’re talking “real world” here, there are other pressures that require trust. Sometimes a supposed expert will have ample time to work, sometimes they’ll need to respond quickly. Sometimes they’ll get second chances, sometimes a single screw up means a catastrophe. Sometimes we’ll get rich data on their work because we’re in person, but often these days we’ll be remote, and just have to... that’s right: trust them.
And if we don’t trust them, we won’t hire them. So they won’t get a chance to prove their expertise (and thus build it, but that’s another story).
Now let’s take a second look at the leading study I mentioned above. It’s very well done by top researchers, and is the first to assess worker performance across a range of potential tasks for someone in that role. In this case we’re talking consultants dealing with various ideation and writing tasks for things like marketing and business strategy. The world and the authors often hold it up as reinforcing the idea that LLMs are a powerful boost to individual performance. Not false. And sometimes we equate that with skill. The consultants who had less skill to begin with see the biggest performance gains using the technology. That’s a real and important finding, obviously.
But its title - “Navigating the Jagged Frontier” - and findings actually highlight the trust problem I’m on about, almost more than they do the productivity boost. That’s because boosts were uneven, and weird. In some cases, users’ performance got worse than it would have been without the tech. In other cases, neutral. The study shows that genAI is an uncertain and powerful tool. On top of that, we do not need to reveal the fact that we’re using genAI to produce our output. From homework to job applications to memos and business proposals, we now know that a huge majority (64%, says the latest high quality survey!) of users don’t disclose they are using this uncertain and powerful tool.
That’s… not exactly the recipe for trust in expertise. In fact, it’s a threat.
Why? We know that experts might be using genAI to help them interpret and intervene in challenges. In fact any time we’re counting on someone for their expertise and they don’t say they’re using genAI, we have to reasonably assume they are, and are willing to pass that work off as their own. This goes way beyond consulting, now that these systems run on our phones and can interpret and produce images. Your plumber or computer repair tech could pull out their phone in the middle of a job. And while that may often be okay, we know the effects are semirandom. We know about hallucination. And, really, what happens if their cell isn’t getting signal in the basement?
At least for now, to place our faith in expertise, we need to trust the human behind the machine.
Getting out of AI check
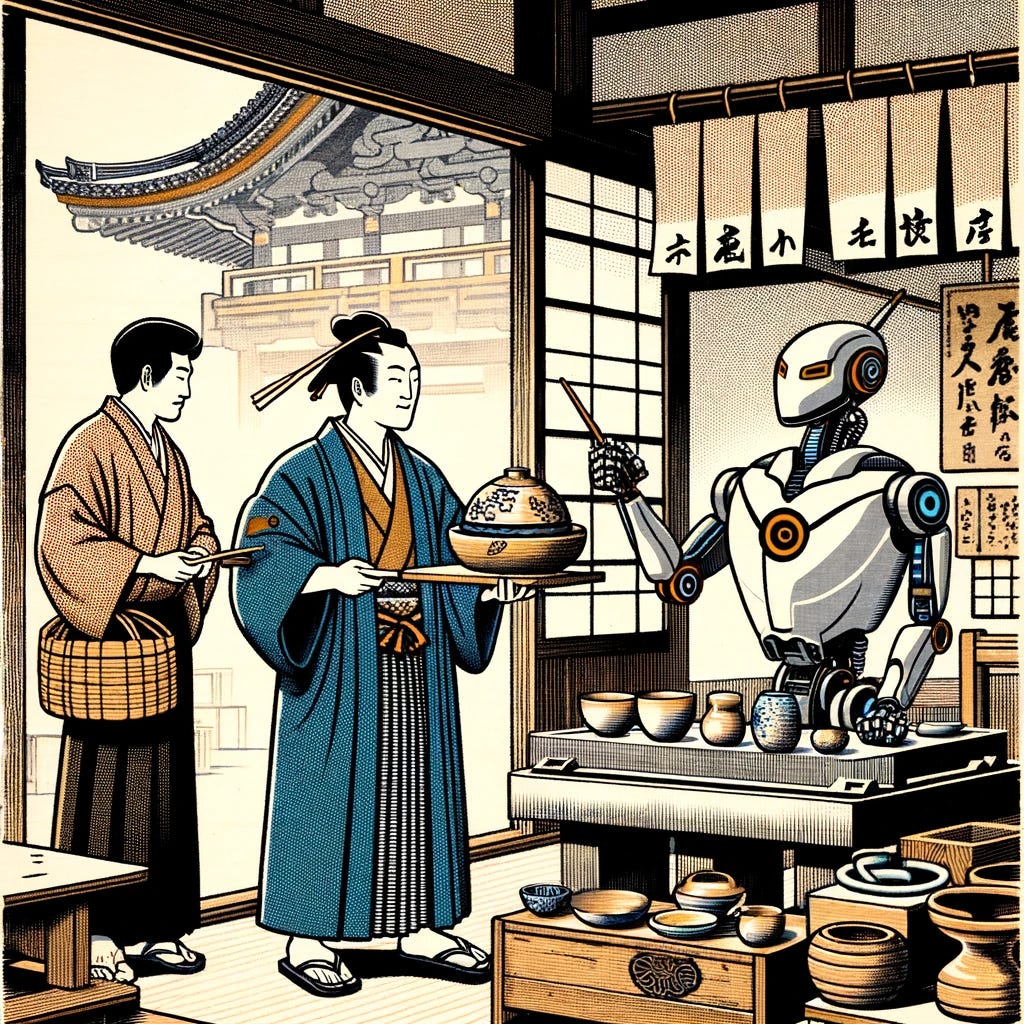
The generic recipe for getting out of AI check is simple: genAI transparency.
Let’s frame this in terms of your expertise.
These days if you want to earn someone’s trust to perform some work, it’s incumbent on you to assure them that you’ve got a handle on this AI thing. That you know your stuff, can get great output from genAI, can tell when it’s wrong, and can do solid work on problems where it’s not helpful or available. And you need to occasionally allow someone to independently audit your work so they can see how “brittle” your expertise is - were you just coughing up what the AI gave you? Were you asking it for a second opinion? For search? Are your prompts suitably sophisticated? The list goes on, and inquiring (client) minds want to know.
This is literally what genAI-engaged professors like me do now with our students. Sure, you can use genAI, we say. We know you’re going to anyway, and we can’t detect it. But the new standard is: show your work by including your full genAI chat transcript with your finished product. If you don’t share a transcript, you’re either really good or you’re lying. And you’ll have to stand and deliver live in class anyway, so we’ll find out. While we’re trying to develop expertise, not rely on it, the need for trust and resulting push for transparency is very similar.
We live in a genAI-turbocharged world, now, and this is the standard that you’re going to need to meet to earn trust in your expertise. Practice AI transparency.
But how to do this, exactly? Just posting your ChatGPT logs online is one way to do it, but ultimately this isn’t very user friendly. Simply saying you use the technology is great, but that leaves too many open questions about your ability to handle unusual or dynamic problems. Potential consumers of expertise need an easy way to assess what you can do now with AI, and how you’ve worked to preserve a suitably broad and flexible range of abilities needed for the messy real world. We need new ways to present our expertise to earn that trust.
One step we can all take: make a genAI portfolio
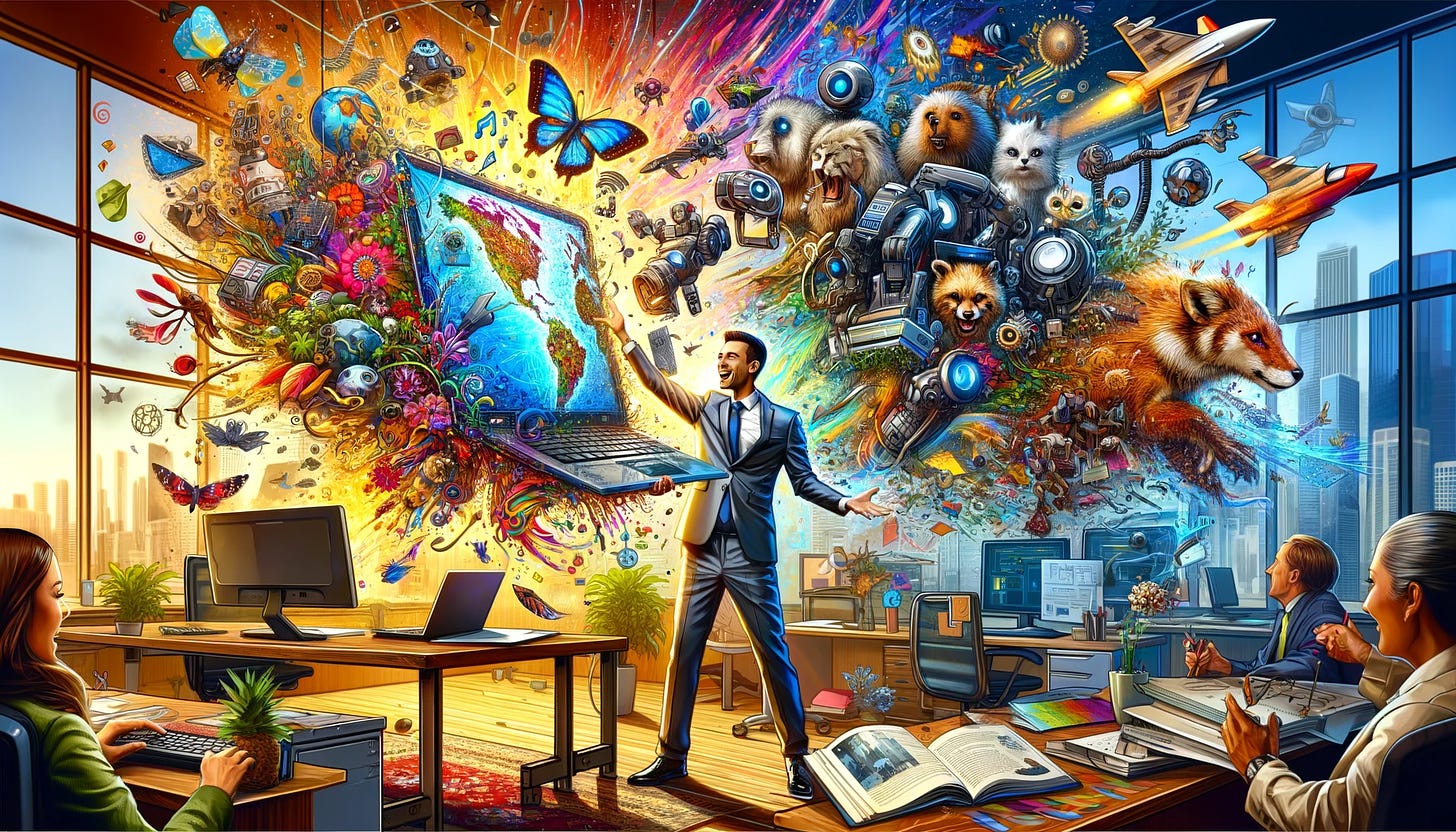
genAI is new. Unrefined, in many ways. It’s still early days. And it has far reaching implications for work across most occupations. That means it’s not an exaggeration to say that perhaps a hundred million working adults are in AI check - right now. That’s a mind boggling array of tasks, situations, tools, and expertise. What’s an efficient way for anyone, in any kind of work, to give some assurance to potential consumers that they’re handling genAI well?
How can we demonstrate genAI transparency?
I’ve got one practical answer for you, and it was inspired by Alexis Chew, an undergraduate student in my “Managing Technology Organizations” course this quarter.
As I wrapped up my second class on generative AI (early in the quarter, because I was going to mandate students use it), Alexis approached me and asked a simple question: “how do we prove our genAI skills to potential employers?” She thought they might value the skills she was building in my class, but that they wouldn’t ask and she didn’t know how to tell.
I said I had no idea, but that it was a great question. I turned it around to Bluesky (the other blue-icon-themed social network), and Jesse Shore dropped in one word: “portfolio”. This seemed instantly on point: the portfolio is flexible genre that is designed to demonstrate expertise for diverse audiences. And Alexis was intrigued - she has diverse skill sets - so we converted a healthy chunk of her course grade into a focused project to produce two things: a research-backed template for a genAI portfolio, and a reasonably fleshed out portfolio for her own expertise.
The first of these documents outlines the range of portfolio types out there, and examines how they work. By accounting for the moving parts inside each type, Alexis was able to propose a few strategies that would be particularly effective for genAI transparency. It turns out, for instance, that “case study” and “narrative” logics seem particularly helpful. All humans understand stories, and cause-and-effect relationships. If we tell stories of a problem we faced, how specifically we used genAI to address them, share the output and its impact, we’re well on the way to helping people understand our hybrid human-genAI expertise and our interests. Alexis, for example, is focused on environmental science and art, and the case studies in her genAI portfolio show how she used this new technology to learn more and get better results in both domains. And in case you’re wondering, yes, she used Bing extensively as she produced both these documents, and submitted her chat transcripts along with them.
She and I are excited to share both, here. Please bear in mind that we view these as works in progress - we agreed it was better to get the ideas out there soon rather than to polish them up for final presentation:
genAI portfolio toolkit (by Alexis Chew)
We hope you’ll adapt, share and use these, and that they inspire many to explore portfolios as a way to account for their expertise with this new set of tools. This may be especially important when we’re dealing with the many folks who are not clear on what the technology is and what it can (and can’t do). And a genAI portfolio should be valuable far beyond the leaving-undergrad-and-seeking-a-first-job category. Been learning genAI and looking for a mid career switch? A genAI portfolio could help underwrite the move. Have a profile on a gig platform like Upwork? This might help you stand out there, too. It can also work as a screening tool for you on the consuming side. If you’re choosing whether or not to join a work group or organization, hire a contractor, or new employee, you can ask: do they have a portfolio of genAI efforts to share?
Trust is a must
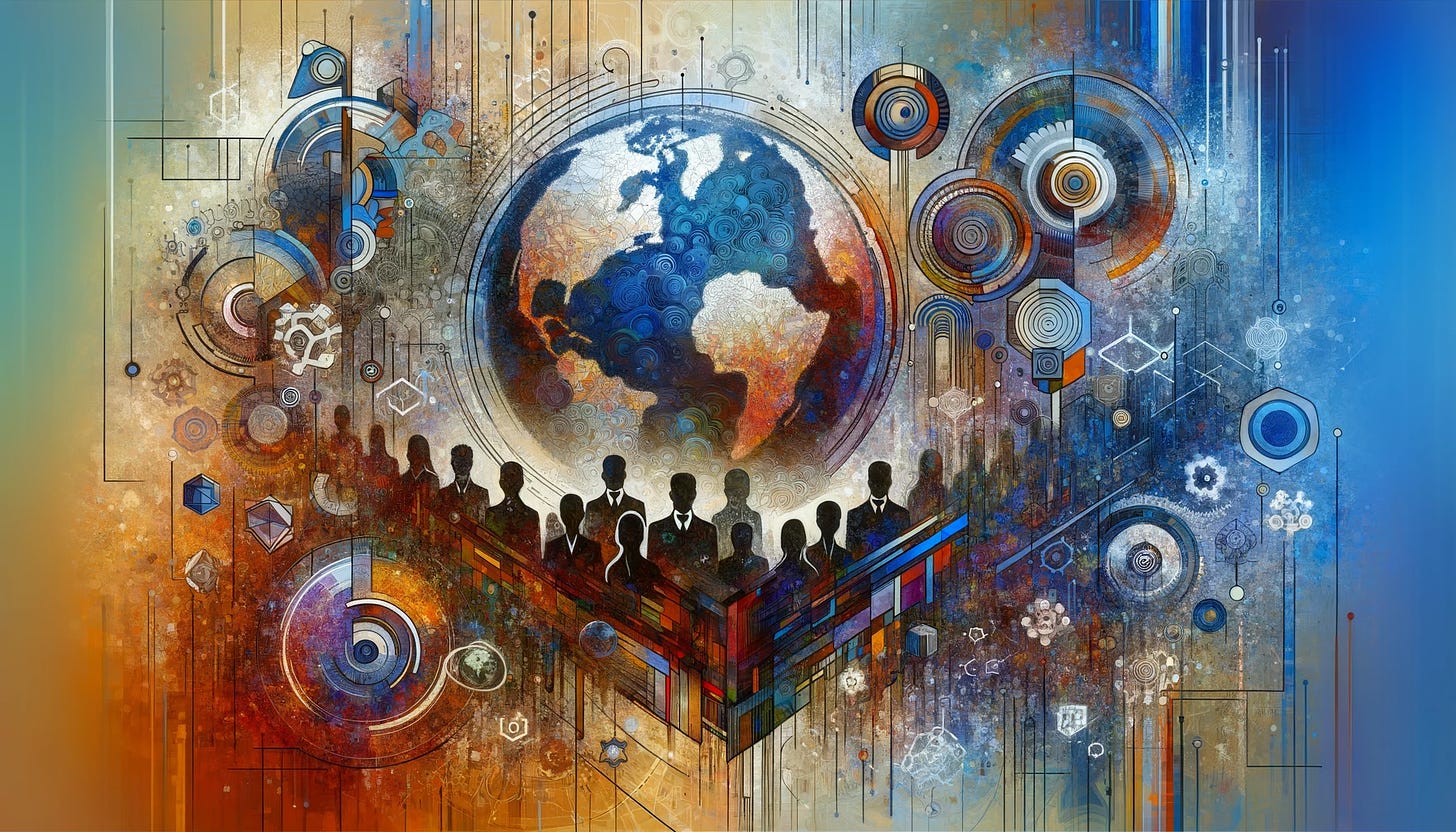
No matter how skillful you are, if people don’t trust you, you won’t get to put your expertise to work. We have seen the arrival of many, many productivity enhancing tools before now - from fire to the potter’s wheel to steam engines to calculators to computers and the internet. None have been quite so broadly capable or “creative” as genAI, while also delivering strangely inconsistent results. And none have been quite so easy to conceal during use. The threats to faith in expertise are unprecedented.
If genAI is useful to you now - or you could imagine it helping you get better results in the future - then people will have new, significant questions about your expertise. Many millions of us are in the same boat. Whether it’s via portfolios or other forms of AI transparency, we will all need to address these gaps in confidence to get permission to put our expertise to work.