How to handle genAI in organizations, according to science
Right now, everyone's gotten into genAI, and that includes organizations. But the three defaults are ignore, ban, and "all hands". These aren't viable or backed by the science. What is?
Been a while, folks. As predicted, I’ve slowed down here a bit to allow for a *lot* of extra writing for popular outlets associated with The Skill Code - my book that comes out on June 11. You can preorder here, and you’re the first to know that - soon - proof of that preorder will allow you to get some exclusive content. More soon there.
Also, I should say that I’ve got… something else I’ll be announcing soon. It’s in the same “space” as the book, quite possibly a FAR bigger deal. That’s also been taking up a lot of my time.
But here’s what got me away from all that: a bunch of well intended, very common, dangerous advice out there about how to handle genAI in organizations. If you or your organization follow this guidance, things will not go well unless you’re lucky. Save those kinds of odds for Vegas - let’s review the science.
But first, to the problematic guidance.
Ignore, Ban, and All Hands
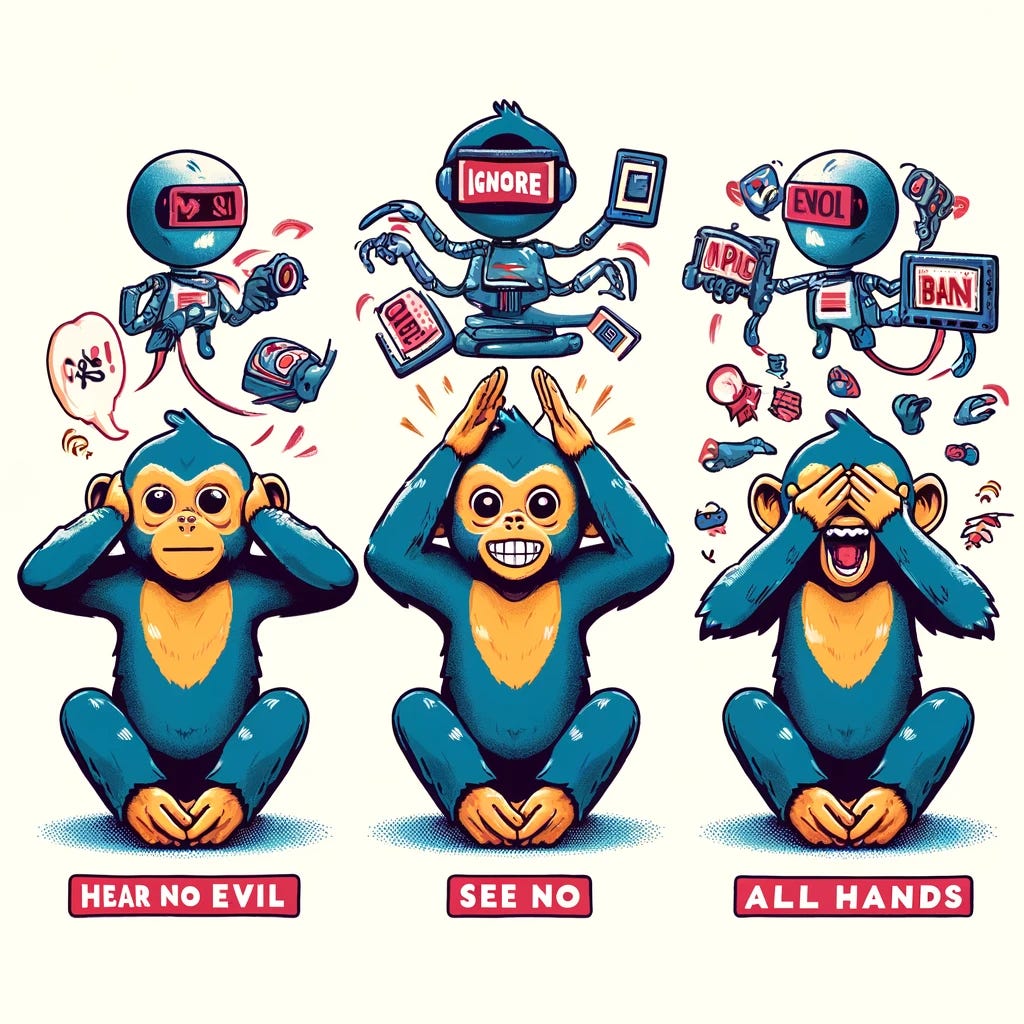
We’ve had three predictable approaches arise in the “how to do genAI in your organization” zeitgeist in the last year.
The first coherent organizational genAI strategy that emerged was ignoring it.
Ask around, and this is still mostly what you’ll find. Leaders at companies might have heard of AI, but they don’t know much about it other than what they overhear as their kids “cheat” on their homework. But - and I mean this seriously - that’s the beginning and the end of their exposure. And their decision making isn’t going to change. If you’re Marcus, the guy (aka research subject) I know who owns and runs a mom-and-pop third party order fulfillment company on the eastern seaboard, you saw a Super Bowl commercial about AI, and you kind of shrugged and went back to trying to get orders, manage costs, and keep your warehouses productive for your customers. It’s a brutal business, and the only time you adopt a disruptive new technology is… never. That’s right. Never. You just don’t. Your margins are razor thin, your customers are hyper-demanding, and your workforce is a dynamic problem. If someone can sell you some technology to solve a real problem, reliably, starting yesterday, you’ll buy it, but the case has to be crystal clear and even then they have to call three times.
More commonly, you identify a need (say a tripling of demand for hand sanitizer during COVID), you realize you can meet it with tools you have on hand (a nail polish filling machine), you find a few more of those on the secondary market… in Canada, and you send a crew up there in a rented truck to pick them up the next day. Digital tech like barcode scanning, warehouse management systems, timecard systems… these are the bleeding edge of what you deal with.
So you ignore AI. In fact, you won’t buy a damn thing with those letters attached, because it sounds unreliable. Artificial? Sounds lower quality. Intelligence? Well, you’re familiar enough with the human kind. Suffice it to say you’d rather rent a conveyor or a spreadsheet than a person, if you can.
The next organizational strategy that emerged was banning generative AI.
We got a vivid, front row seat on this one in education, and many of us still have it. Teachers and administrators quickly got wise to the fact that students could input their homework into Bing or ChatGPT and get B+/A- material out in seconds. And its range was dizzying - everything from creative writing to history, coding to complex word problems, book reports to resumes. They concluded - accurately, in many cases - that unrestricted use of genAI on homework would destroy the learning value in those assignments. Struggle, careful thought, mistakes, and useful feedback are the hallmarks of the learning process, and all of this could get shortcut by a few keystrokes. This looked like an existential educational threat, so…
Many schools banned the technology. Entirely. Part of why they moved so swiftly in this direction had to do with the illusion of enforcement - plagiarism and cheating detection software had gotten quite good for previous, specialized problems, and administrators and teachers convinced themselves that these would do just as well at detecting generative AI use. We knew within weeks that this was patently false, but the belief and the practice of detection (and subsequent punishment) continues. Some teachers and administrators probably know this and just tell their students that these technologies could sniff out generative AI output, hoping the fear of punishment will curb its use.
Regardless, a ban remains. And while it doesn’t get nearly as much press, many companies have chosen the same path. Do not use generative AI, the word came down. Cheating was less the concern there than security - each time you prompt an LLM, the company that made it gets that data, and all of your responses in the prompting chain. And if that prompt includes company proprietary data, source code, or other competitively sensitive information, well… you’ re giving it away, and you’re probably fired. At a banning company, anyway.
A secondary concern here is the now well-known “hallucination” that genAI engages in. These systems produce very convincing output, and some of it is utterly made up. Yet human users can’t tell, have a strong incentive to save time and boost quality, so they accept that output and pass it along to those who will rely on it in some way. Then… oops. We all now know the story of the lawyer who wrote and submitted a brief using ChatGPT and got found out. But what, managers wonder, about the silent majority of users in my company who will do the equivalent but not get noticed? That’s like a creeping quality plague throughout the firm, one that no one has an immediate incentive to notice or remediate. What to do? Ban it.
The third strategy is “All hands”. This has only just emerged in the last few months, and is largely a reaction to the first two (and of course the broadening realization that we really have invented an imperfect, powerful, general-purpose cognitive prosthesis). For many leaders, managers, and business owners, inaction or resistance is clearly not an option - the only question is about how to engage. It’s also clear to those folks that no matter what they do their employees (or students) will use genAI to do their jobs, whether it’s via their smartphones or their work computers. They also know it’s not cost effective to try to detect this. And maybe they’ve gotten around to listening to or reading Ethan Mollick - that Wharton professor who seems to know all about it earlier than everyone else. Maybe they got Co-Intelligence, his new, NYT-bestselling book, maybe they found OneUsefulThing, his substack, or maybe saw him in the Wall Street Journal, or whatever.
Ethan lit a fire under everyone’s chairs - do nothing and you will be left behind. This is evident right out of the gate with his four principles for dealing with genAI. The first and foremost - the one he put in pole position for emphasis - is “Always invite AI to the table.” Ethan tells us all we should be playing around with this stuff. As in everyone in your company. Right now. Because nobody quite knows what genAI is good for, and those organizations that figure it out quickly are going to win. Just like kids did in education, your employees are figuring out new hacks with a new general purpose technology, and you had better steer into the skid. Deeper into that same chapter in his book Ethan pulls back from this a bit, suggesting that leaders should get help from “their most advanced users” and offer incentives for innovation. That’s better, but it’s buried.
Whether they were influenced by Ethan or not, a new class of leader and manager has emerged - one hellbent on granting full, paid, open access to LLM-based chatbots to every employee in the company, and telling them all some variant of “we need to learn how to handle genAI together”. Announcements go out. Enterprise-level OpenAI subscriptions get bought. And the organization lurches towards the technology, maybe faster than it’s lurched towards anything in recent memory. Hard not to hear the words “ChatGPT” or “AI” in the halls, the bathrooms, or on videoconferences. In fact it’s hard not to interact with genAI in these contexts - many folks get genAI-generated summaries of their online meetings for instance, complete with action items and participation feedback, for example. In this organizational mode, genAI is the new electricity, and it’s always on.
Science says: none of these are good enough
First, let me acknowledge that all of these organizational approaches have significant strengths, and will probably save the day in isolated instances.
What? Ignoring and banning genAI might be ideal?
That’s exactly right. I wrote about this a few months ago in “AI is fast, Automation is slow - Can they meet?”, so if you want a deeper dive into the research on automation and new general purpose technologies in organizations, you can head over there. But, tl;dr: most of the time, going early and fast with new, disruptive technologies does not end well for organizations. A few succeed, but most will hurl themselves at the problem in the wrong ways, or at the wrong times, and they will spin their wheels, burn money, and harm people (sometimes literally). The thing is we have no research (that I can find) that shows the organizations that survived or even thrived because they did not hurl themselves at the tech, or that shows how frequently this is a winning strategy. The closest we come on that score is the research on “fast following” - in other words waiting for someone else to go first, learning from them, and going second. But the term “fast” there should be a dead giveaway - there’s no “slow following” research. We instead study successful adaptation, showing that it takes a while, proceeds through experimentation and dramatic investment, and so on. That research bias reverberates through business-school-style academic advice on what to do - and vigorous action is the core recommendation. Ignoring new tech or even banning it aren’t in the playbook, even though those are likely better bets for many, at least for a while.
But that’s not to say that “all hands” is all wrong. It will save the day for some organizations, too. Especially when the cost and pace of experimentation is low and the technology is new, a huge swathe of research on technology and organizing tells us that if management adopts a new technology, rank-and-file employees will rapidly find ways of slotting that tech into their work, find new failure modes and risks, and incrementally adapt things to suit. The process is often messy but can get good results, providing employees at all levels with useful intel to guide decision making. And we have great research that shows that firms that invest in advanced automation like robotics tend to outcompete firms that don’t. Firms that invest hire more people because they are more productive, can grow as a result, and take customers from other firms. It’s those other firms that shed jobs.
But any savvy manager - or employee - needs to face facts: all three of these approaches are hamfisted. You wouldn’t try to eat a four-course meal with just one utensil, and if you take any of those three approaches above, you’re doing the organizational equivalent with genAI: ignoring a mountain of superb science on innovation, technology and organizing and organizational change. Put together they offer a far more nuanced, but clear, and tested organizational guidance that will greatly increase your odds of success with genAI.
Science-backed genAI implementation, ftw
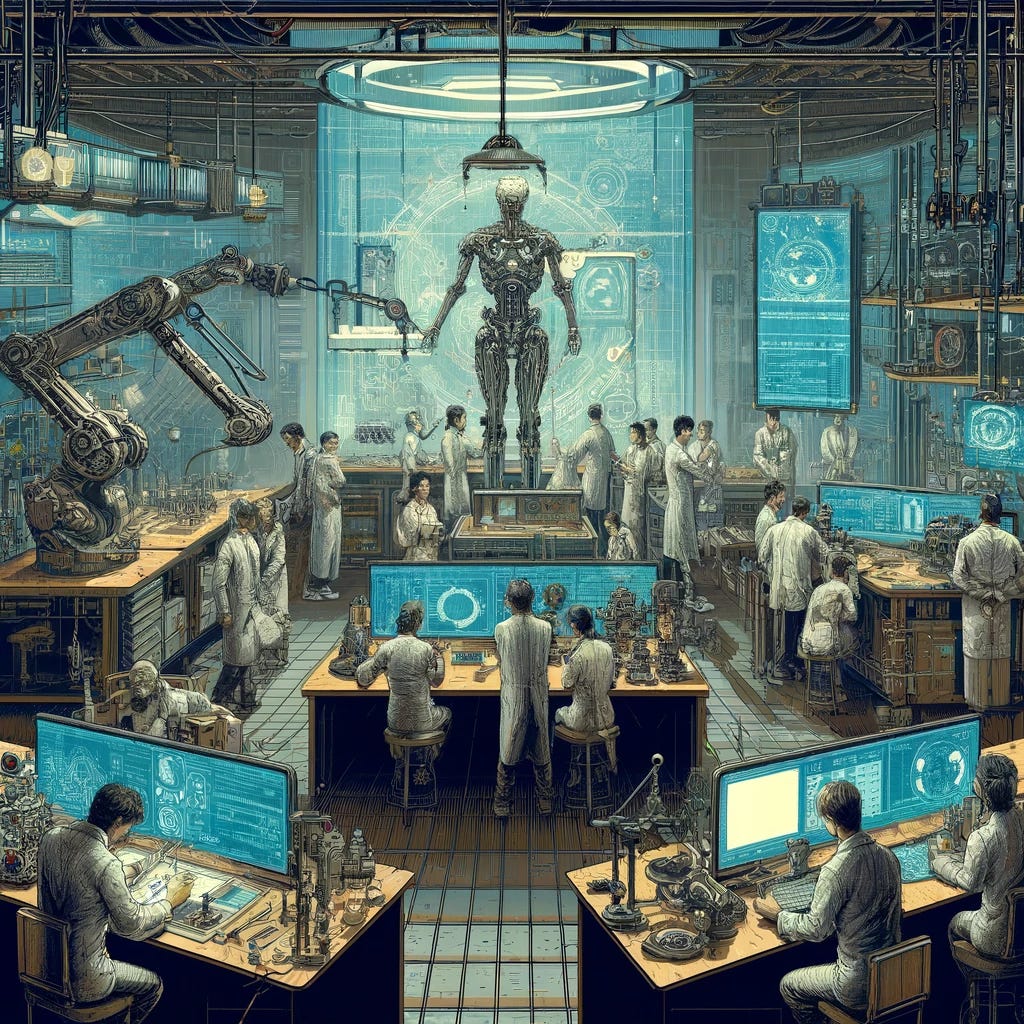
The first core insight is that a lot of the tactics that help an organization adapt to disruptive new technologies get implemented outside that organization. That’s an invisible dimension in the three tactics above - they’re all done on the inside. So, lesson number one, if you don’t want to hobble your efforts: you’re we have to watch for tactics that apply on the inside and the outside. The other critical dimension that’s muddled together above is the individual/collective one. Some tactics apply to groups of people, while others are much more atomic, having to do with individual humans at work. Lesson number two is be sure you’re choosing the right activity level for the tactic you’re choosing, and don’t leave good options off the table.
The second you parse the field this way, then take a look at the research, a host of targets and tactics pop out. I’m not going to provide an exhaustive list here - just a few in each category to get you thinking. The core message is: effective organizational adaptation to truly disruptive technologies is liable to require multiple approaches drawn from all four squares, and the savvy manager will go hunting through the research to find those that fit.
So here’s that 2x2:
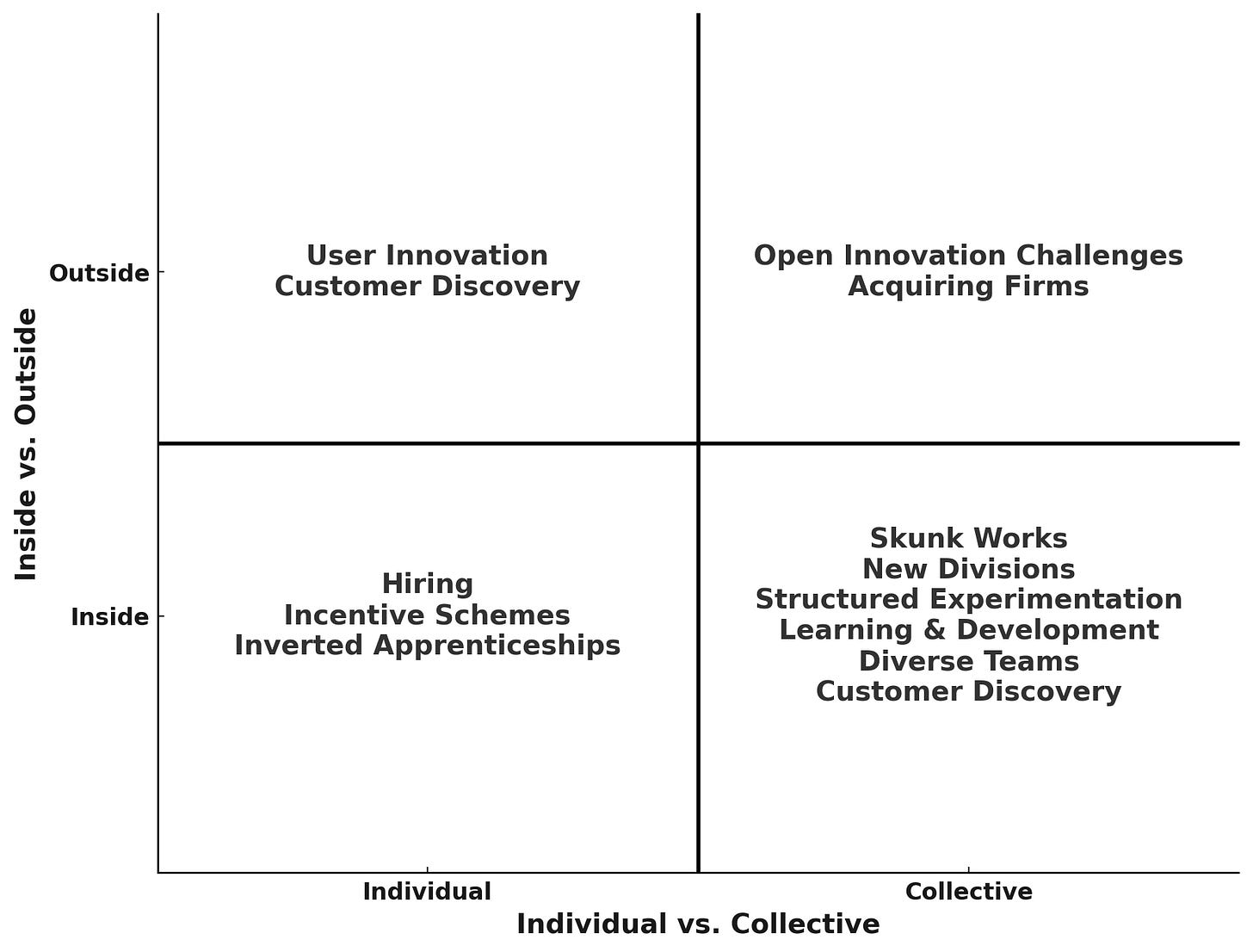
A detailed breakdown of even this subsample of tactics - and their relationship to organizational adaptation to disruptive technology - would take way more space than you’d allow, and wouldn’t be all that fun. So I’m going to buzz the tops of the trees here, give you a few links to relevant research.
Collective, Inside
Skunk Works: When it’s time to pivot or try something new, special teams get formed within organizations, often isolated from normal operations, to innovate and develop new technologies rapidly. These teams operate under the principle of autonomy and minimal bureaucratic oversight, which fosters creativity and speed. Just got confirmation last week from an AI researcher in the mobile space that only just now (i.e., way too late) a big tech firm has set up a skunk works team and dramatically throttled their compute so they are forced to be efficient on mobile. That’s the idea. Reference: Skunk Works: A Personal Memoir of My Years at Lockheed by Ben R. Rich and Leo Janos (1996).
New Divisions: This is perhaps the most well known, best researched play: creating dedicated new divisions within the organization focused on leveraging disruptive technologies, allowing concentrated expertise and resources to adapt and innovate effectively. And yes, this means a new offering to the world, not a new internal function. This requires dramatic capital outlay, which means sacrifices in other areas. Reference: Christensen, C. M. (1997). The Innovator's Dilemma: When New Technologies Cause Great Firms to Fail.
Structured Experimentation: This is perhaps the most important way to channel rank-and-file, individual experimentation with genAI at work so that productivity sustainably improves. This involves setting up controlled, systematic experiments to test hypotheses about how new technologies can be integrated and utilized within the company. Toyota is famous for a *fantastic* method here - called the A6 - that I helped Nelson Repenning teach to executive MBAs at MIT’s Sloan school. He and his collaborator Don Kieffer (former Harley exec) refined and improved it. If you’re looking for a “color by numbers” approach to getting organizational value from new technology, this would be it. Reference: The Most Underrated Skill in Management by Repenning, Kieffer, Astor (2017).
Learning & Development: It’s so subtle and utterly urgent that I’m hitting it again - last month I wrote “Learning and Development: The New #1 Organizational Function.” This goes way beyond formal training or even job aids or job rotation programs. In fact those are dangerous wastes of time in many cases right now. They’re all centrally-driven, and now the game is learning from your employees as much as helping them build capability and learning from each other. Humans don’t learn any differently than they used to, but we’re in the middle of a practically discontinuous change in the technicum, so L&D has a far broader mandate. Reference: Is Yours a Learning Organization? Garvin, Edmondson, Giino (2006).
Diverse Teams: Research is very clear on this - when discovery and invention are paramount, teams with strong cognitive diversity outperform those that are homogeneous. So beyond doing things liek cultivating psychological safety (obviously critical) Leveraging the diverse perspectives within the organization to foster innovative ideas and approaches is essential for adapting to and integrating new technologies. Reference: Why Diverse Teams Are Smarter by David Rock and Heidi Grant (2016).
Collective, Outside
Open Innovation Challenges: Yes, an organization can - and sometimes must - engage with collectives outside its borders to get the right new idea about how to approach its reality. Open innovation is one key way of doing this, and involves leveraging the knowledge and creativity of external contributors through collaborative partnerships or competitions to solve problems and develop new technologies or products. The classic example here is NASA getting a new solution to predicting solar flares from a retired radio systems engineer, but your organization could just as well run an open challenge about how to put genAI to use. Reference: Chesbrough, H. W. (2003). Open Innovation: The New Imperative for Creating and Profiting from Technology.
Acquiring Firms: Sometimes, it’s just flat out true that no matter how hard you try to adapt to a disruptive technology within your organization, you can “learn” faster by simply purchasing or merging with another firm that is getting there faster. They found the right mix of talent, tools, techniques, and other resources to get the job done - why reinvent the wheel, even if you could? For those outside this research tradition, this is a surprisingly common way for large firms to manage the challenge of changing with the times. Reference: Rules to Acquire By by Bruce Nolop (2007).
Individual, Outside
User Innovation: Eric Von Hippel (a former prof of mine) pioneered research on this incredible phenomenon - many times, it’s individual users out in the real world who invent at least the first version of new technologies, products, services, techniques or blends of these things. It was an EMT mountain biker who invented the camelbak, the now ubiquitous sack of water you wear on your back - he filled an IV bag with water, slapped a tube on it and tied it around his back for a race. Von Hippel has since made a playbook for finding these users and integrating their early discoveries back into the organization’s offerings. Reference: User Innovation (open MIT courseware, by Eric himself).
Customer Discovery: Somewhat akin to user innovation studies, this involves actively seeking insights from individual customers outside the organization to guide the development and refinement of technologies. Organizations do this all the time to learn what to build next. Why not do it to find out if your customers have found a killer genAI app? You might find one for yourself, and find a new problem you can solve for them in one fell swoop. Reference: The Four Steps to the Epiphany: Successful Strategies for Products that Win. Steve Blank, 2013
Individual, Inside
Hiring: Organizations hire all the time. Why not focus your hiring on talent that can help with your genAI problem? I don’t mean machine learning engineers here, I mean people who - in addition to having the qualifications for their job - know a thing or two more than most about how to put genAI to work. This fall, Alexis, an undergrad of mine, worked up a first-in-kind genAI portfolio to help potential employers see this more clearly. If folks follow her lead, this kind of hiring will get easier. Reference: The Hiring and Firing Question and Beyond by Barbara Mitchell.
Incentive Schemes: Ethan Mollick has rightly drawn attention here. Offer genAI bounties! Or status boosts (A title change, modified responsibilities, and/or increased visibility?) Managers know a lot about how to set up reward systems that motivate individuals - now just focus these on experimenting with and adopting genAI in ways that also help the organization. Doing incentives right is hard, but research is pretty clear on how to avoid common pitfalls. Reference: When Your Incentive System Backfires by Vijay Govindarajan and Srikanth Srinivas (2013).
Inverted Apprenticeships: Fine, this one’s mine - with ace ethnographer and coauthor Callen Anthony at NYU Stern. In our original research, published just last August, we showed that when new technologies arrive, senior experts can rearrange their working relationships with novices to “mooch” off them as they engage with new technologies. Unfortunately this is rarely a winning equation for the novice in the wild, but we found one clear approach to this that left the novice and the expert better off in their skills with the new technology. Reference: Inverted Apprenticeship, Matthew Beane and Callen Anthony, 2023.
Now, work it all into a plan
So fine, there’s an incomplete menu of scalpel-like tactics to carve out an elegant genAI learning journey for your organization. Given that 2x2 you can go find more that suit your situation.
But that’s not enough. Just having the right instruments on the tray doesn’t mean you can get to work: you need a plan. Each of these tactics needs to be slotted into a coherent process - where each step is more valuable because of the results from the previous one. There, my colleague Paul Leonardi wrote a Harvard Business Review article last year introducing his STEP framework. Here’s a direct rip from his piece:
segmenting tasks for either AI automation or AI augmentation;
transitioning tasks across work roles;
educating workers to take advantage of AI’s evolving capabilities and to acquire new skills that their changing jobs require; and
evaluating performance to reflect employees’ learning and the help they give others.
Hard to do any better there - if anyone on the planet can synthesize five decades of research on how technology actually gets implemented in organizations, it’s Paul. And he tuned it to generative AI in 2023! Way ahead of schedule.
A complementary resource here is a solid understanding of how change happens in organizations, and how leaders can foster it. Organizations are built to deliver reliable results on known problems, and inertia (i.e., “best practice”, culture, etc.) will keep them trying to do that even in the face of an existential threat or discontinuous opportunity. They have to get woken up, motivated, see improvement, then settle back down. John Kotter’s 8 step process has been the platinum standard here for decades. I dare you - look at a real change that stuck in an organization - a change that mattered - and you’ll see these steps behind it.
So the next time you see blanket ignorance, bans, or all-hands frenzy in response to genAI - or get pressure to head in these directions, trot out this 2x2, find the right tactics for your situation, string them together in a healthy sequence, and get to work helping your organization adapt. No guarantee of success, of course - these are discontinuous times - but at least you’ll be sitting down to dinner with a full set of utensils.
ps: everything above is false.
Sort of.
Why? genAI itself.
The logic here has a creeping inevitability: we can use genAI in prior tasks, and that changes how we do them and the results we get. So… all those tactics can be performed differently with genAI in the loop. Sometimes to the tune of improved or degraded results, but other times to the tune of results we couldn’t get before - that we didn’t think were possible. How? Nobody knows. Seriously.
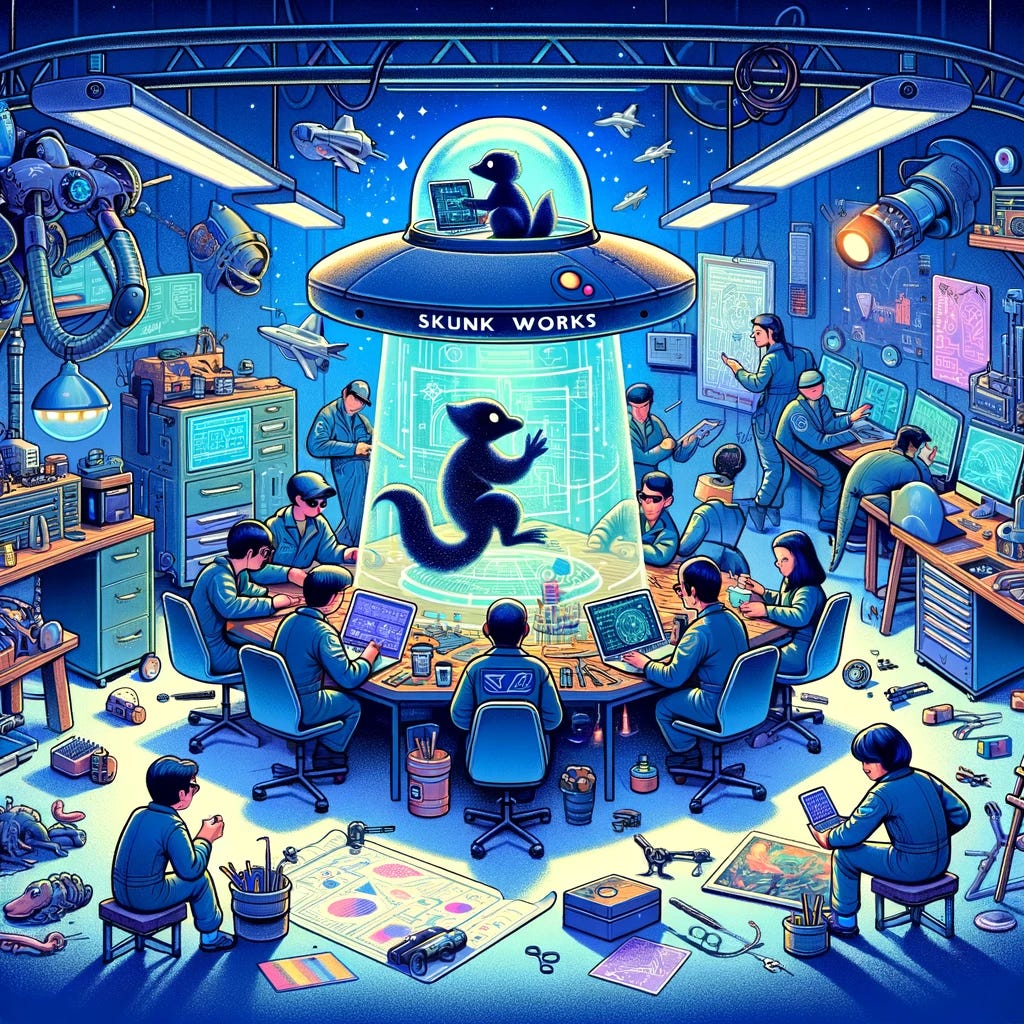
Some individuals, groups, and organizations out there are finding genAI-infused ways of doing skunk works, incentive schemes, open innovation, and customer discovery. But those successes are partial, at best, and nobody (as far as I know) is studying them - even inside the organizations in which they’re being discovered!
This is why I waved a big red flag around L&D as a critical function, and why I, Ethan, Paul, and others are saying all bets are off, unless we experiment with new ways of working.
So go ahead - turn to the traditional playbook that can fill out that 2x2. It’s certainly better than the alternative. But be on the lookout for surprises. Deviance. Nonsensical stuff. It may well unlock a new way to help organizations learn in this age of intelligent machines.
Excellent thought provoking piece. In my experience working with dozens of companies evaluating how, when and where to use GenAI, once we get past the "will it work? can I trust it? will it embarrass me?", we focus on the transaction economics like any tech implementation: What KPI are you try to change? What is it now? What do you want it to be? By when? and then do the math. Does your idea of using GenAI help? does it help enough? If you have 60,000 customer service calls a week at $13.75 per call, and GenAI can deliver quality and reduce that by 15%, it's a simple business decision to do give it a shot. It is actually much much harder to get the money to invest, the people to build and test, and the adapt adjacent processes, get them all on-board at the same time. Then you have to pick and integrate the right tech solution into your current systems. Many ideas presented are just bad ideas because you do something new that is not actually really needed and does not impact above KPIs: "who thought this was a good idea?" (the committee, not the end users) or "great but that only saved me 3 minutes this week." (Not interesting enough).
Great post. This maps to much of my thinking, and in fact I'm noodling on a similar post right now. Your point about thin margins in small enterprises is an underappreciated one. Many of the salivating venture capitalists and technologists who think that AI is going to take over everything all at once don't really seem to understand how non-tech non-venture scale companies operate or the constraints under which they operate. Hilarity ensues when startups try to sell into these companies.
The smartest operators I have seen in this space pursue AI applications in one industry vertical. They get close to their customers, they intimately learn these customers' problems and pain points, and they build an AI solution to solve that very specific problem. AI tech is, in theory, a very generlizable technology but it is not yet generalizably plug and play like, say, electricity.