Take a deep breath: no need to rush on AI
Experimentation is usually wasteful, and easy-to-use AI apps are on the way.
Listening to geeks like me can get you stressed out on AI.
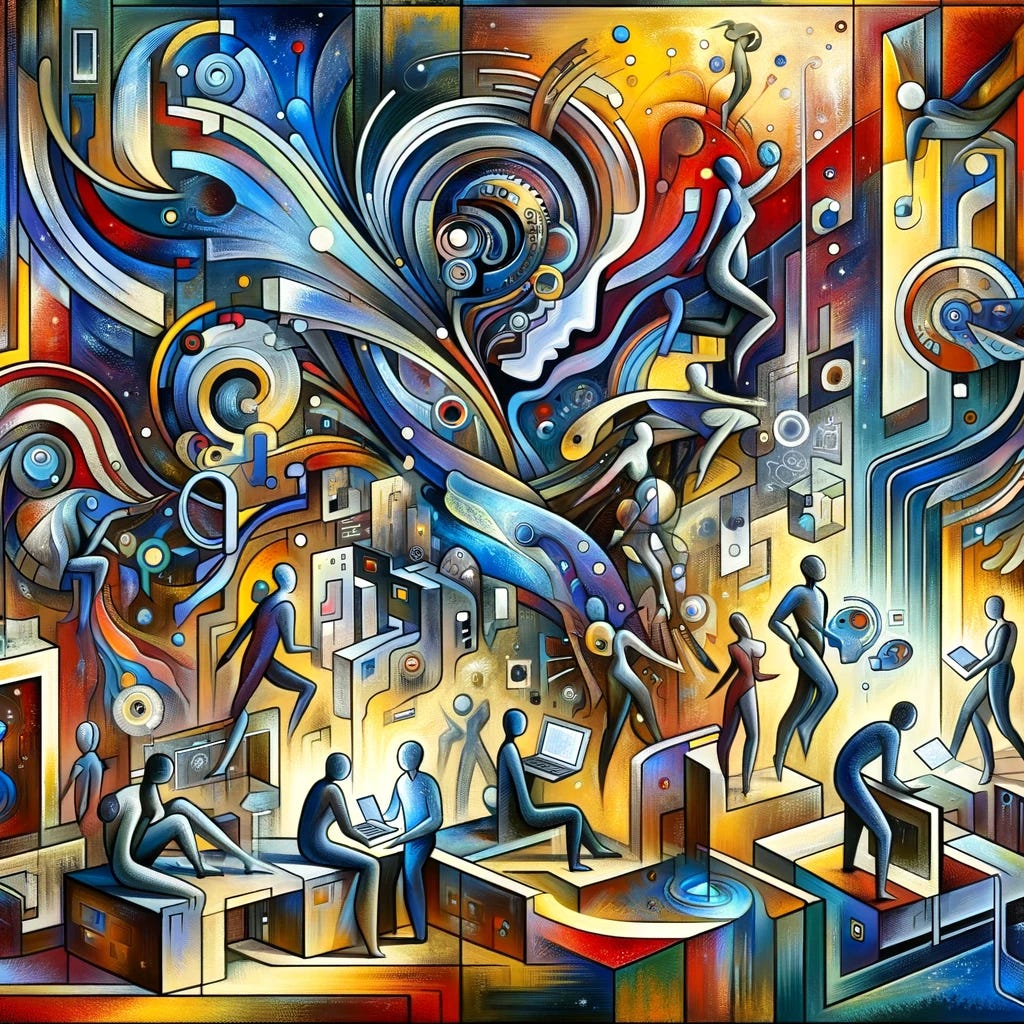
It’s moving FAST, and no one knows what’s next. Sam Altman’s out at OpenAI! Wait, no, now he’s at Microsoft! Wait, no, he’s back! Is all this because they had achieved AGI (artificial general intelligence, aka the holy grail) and the board thought Sam wasn’t being safe enough with it? Or is it because he was going too commercial? Does it matter? And that’s to say nothing of Google/Alphabet’s looming announcement about their ChatGPT buster, Gemini (coming Q1 2024, we’re now told). Microsoft is spending nearly 14% of its total company capital expenditures on AI, apparently twice as much as Google and Meta, and nearly four times as much as IBM and Amazon Web Services (AWS). And finally, without ever using the words “generative” and “AI” in the same sentence, Apple is reportedly investing a billion dollars a year in generative AI to preserve its position.
And non-tech organizations are following suit. Price Waterhouse Cooper has announced a billion dollar investment in generative AI technology, saying it will transform its organization - and workers’ jobs - as a result. Visa has announced it will invest $100m in generative AI possibilities for financial transactions. Khan Academy is well into its deployment of KhanMigo, a supportive chatbot for millions of students and teachers with generative AI under the hood. This may run fast, far, deep, and wide: if some commentators are to be believed, every healthy organization with even a modicum of data will spin up its own “enterprise LLM” to gain competitive advantage. And for now, governments are pretty quiet about any limits to this techsplosion.
There’s sound, there’s fury, and… there’s blistering progress on a new computational paradigm that is almost alien compared to what we’ve all become accustomed to.
Where does that leave us?
You might be thinking: When do I use search, and when do I do prompting? And what IS a good prompt anyway? How do I write one? Shouldn’t I be learning to code with ChatGPT by my side? Or programming up one of these new GPTs to… do things for… someone? And, sorry, hang on, what even is a GPT again? And where do I do all this - is it ChatGPT, Bing, or what are these AI-enabled search results I see in Google now? Is that the same? I’m not spending hours a day on this stuff, and when I try it it just kind of is… okay? Sometimes even a waste of time. Am I doing something wrong? Am I going to get left behind?
Yes, you will be left behind
From one point of view, a small number of people and organizations are racing ahead with generative AI, and most of us are stuck in the past. If you don’t jump, you lose!
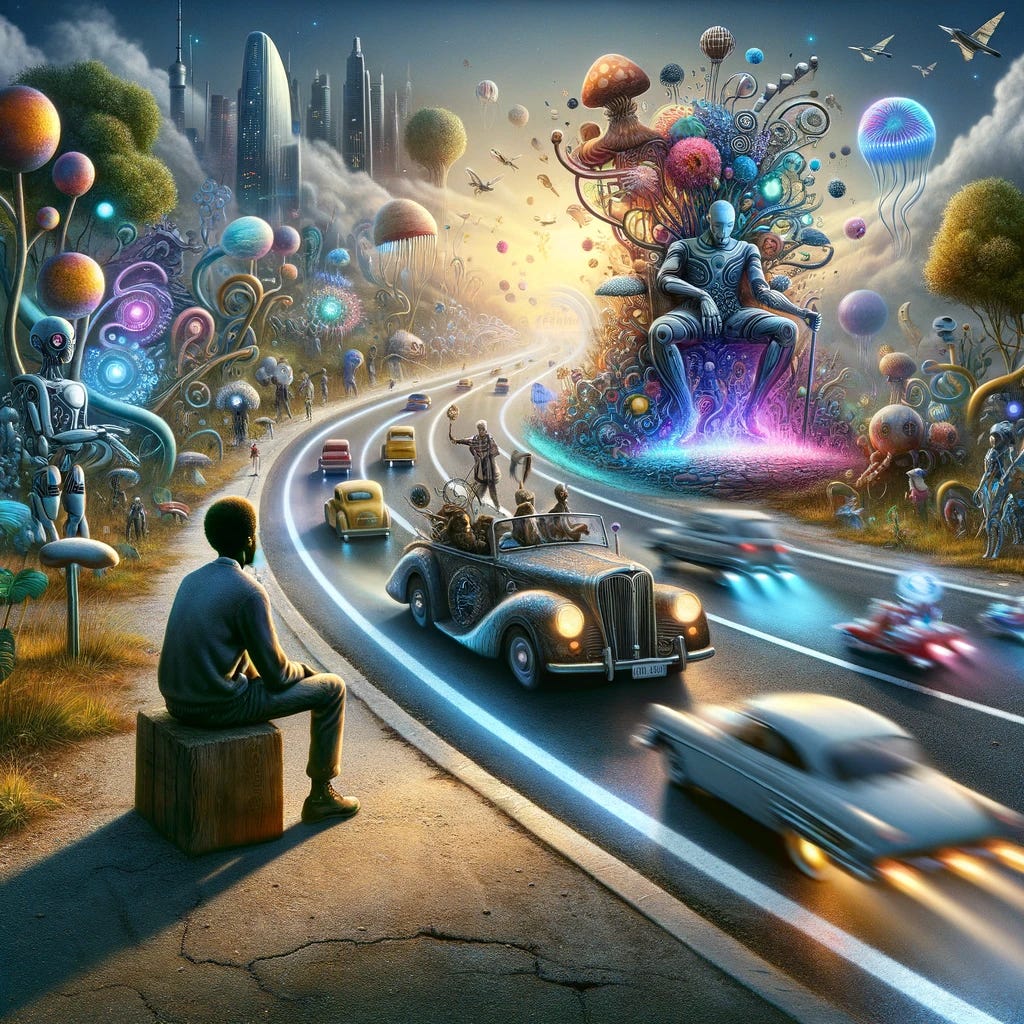
To see this dynamic we need reach no further than the hot-off-the-presses working paper from a star-studded team at Harvard, Wharton, MIT and Boston Consulting Group. The authors and the news media make a lot of the “twist” in this paper: consultants with lower skill get a bigger performance boost than those who already had high skill. That’s an interesting and important finding, but focusing there has meant we often breeze right by the jaw-dropper - here, straight from Ethan Mollick’s substack: “Consultants using AI finished 12.2% more tasks on average, completed tasks 25.1% more quickly, and produced 40% higher quality results than those without.”
We have decades of research showing similar dynamics - for individual and organizational productivity. Clark Gilbert’s research showed this for the advent of the internet and the news industry. Most players froze, or moved slowly. Those who pounced on the internet leapt ahead. Don Sull showed us that when radial tires came along, Firestone Tire & Rubber responded by accelerating activities that contributed to its prior success. A few hospitals in my nationwide research of robotic surgery did the same: they refused to use robots and doubled down on “open” and “lap”(aroscopic) methods. And recently, we’ve been getting studies about the deployment of robots, showing that the firms that invest early get significant productivity gains, hire more people, and their competitors (who don’t buy many - or any - robots) lose marketshare and shed jobs.
The key link between organizational failures and your skills and job is sitting right there in those studies. If you’re not in an adopting firm, you don’t get to use the new tech, so you won’t get healthy opportunities to build skill with it.
We can extrapolate this to the atomized world of work today: all of us have always-on access to the same, top-of-the-line automating technology. Every freelancer, knowledge worker, and student has a strong productivity incentive to use it. Many of us will try, and some of us will race ahead with it. This is what Erik Brynjolfsson means when he says “workers who work with generative AI will replace those who don’t.”
In case you’re now firmly convinced that you’re in trouble - that doing your job as you always have means someone out there gets these kinds of immediate boosts from using genAI - I have great news.
There is a giant hole in this argument, wedged open by decades of research. One you can coast right through without lifting a finger. You might even end up ahead as a result.
Most early adopters are going to pay… dearly
The truth is that most of those who try to race ahead fall flat on their faces. We don’t study them much. We focus on winners.
A classic, clean example of this comes through “The Computer and the Dynamo”, a marvelous study of the adoption of the dynamo by economic historian Paul David. The dynamo - a revolutionary, general purpose power source compared to steam - became commercially available in 1880. Yet David reminded us that manufacturing productivity only took a notable bump by about 1920. The point David makes in the paper is that improvements come very slowly we need to change organizations - work processes and infrastructure - to take advantage of new general purpose technologies. And that kind of change takes a lot longer than just buying the new technology.
But without intending to, David makes an even deeper point. One you can get at just by asking what was happening with dynamos in industry from 1880 to 1915 or so.
It wasn’t nothing. Early adopter businesses everywhere were trying to integrate dynamos into their operations in one way or another. They failed. For at least 20 years - and more likely 30 - they wasted money, interrupted operations, and most likely caused economic, emotional, and physical harm to their workers. A primary failed approach, David shows us, was to replace their steam engines with dynamos. The problem there was that most factories had a single, giant steam engine in the middle of the building. This engine turned a giant shaft, which had numerous belts attached to it. Those belts distributed power to equipment throughout the building:
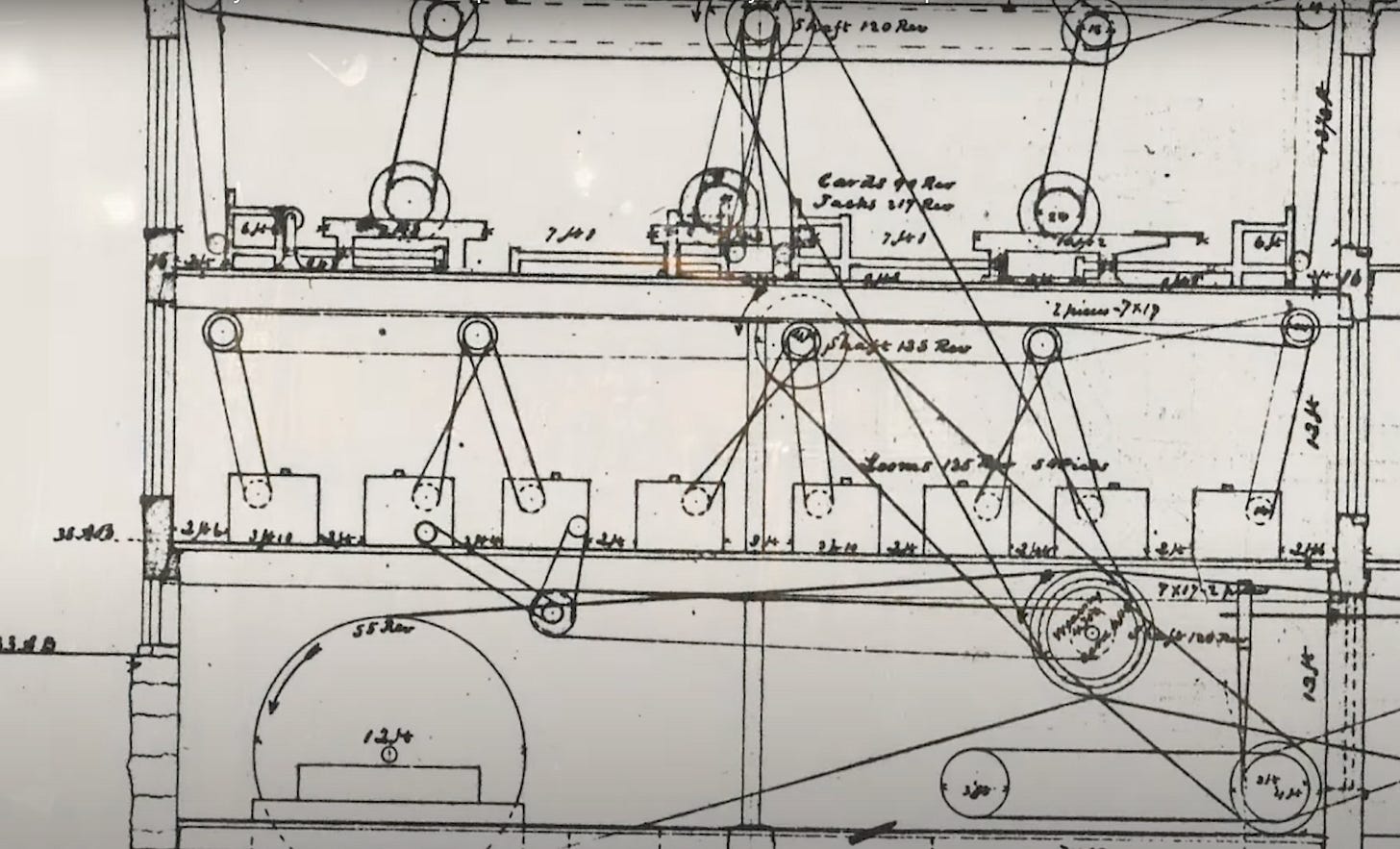
Firms just swapped out the steam engine for a giant dynamo. They kept the belts in place, because replacing those would be very expensive. But also because they couldn’t imagine another way of handling the power distribution problem. The ultimate solution was to put many smaller dynamos throughout the building, which allowed for more flexible manufacturing operations. But the deeper point here is that getting there meant many factories and firms burned significant capital, time, and employee welfare trying this technology out - and most of them failed.
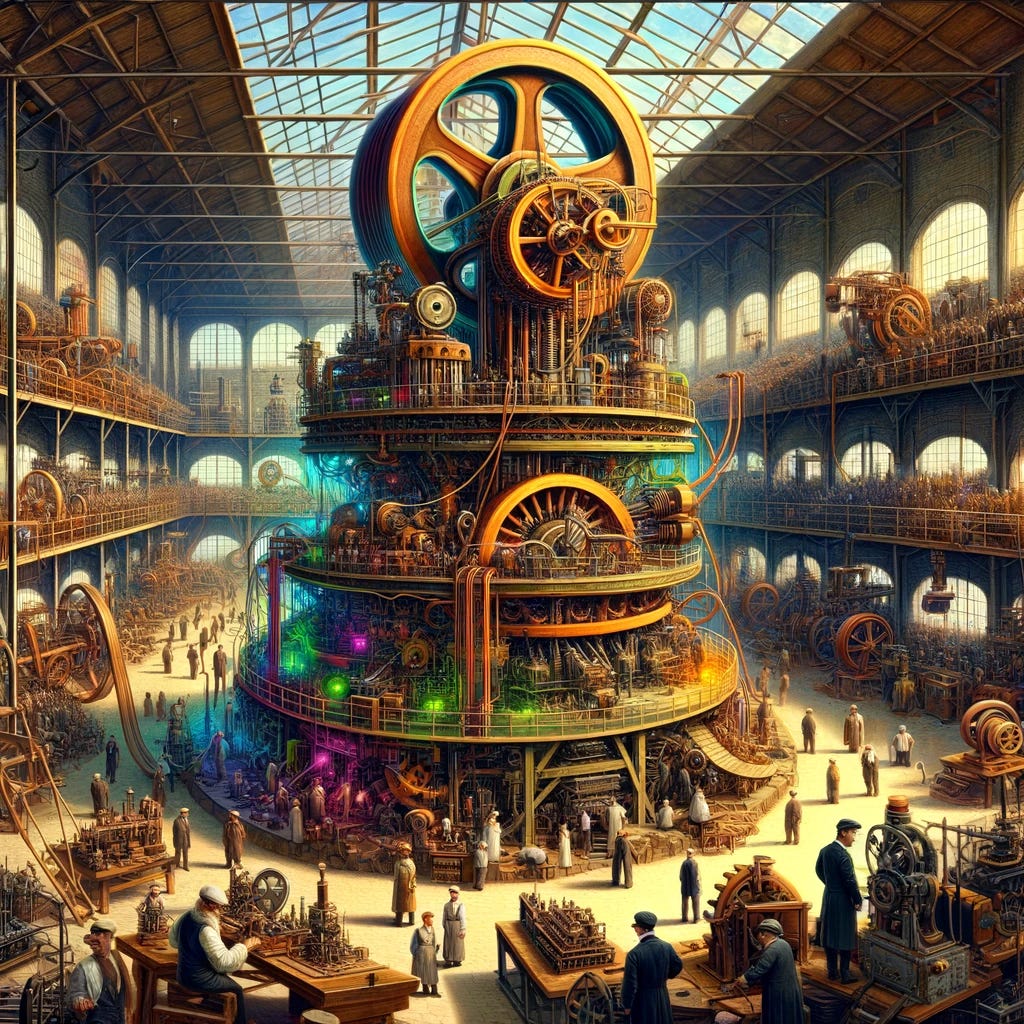
History doesn’t record these failures. All that waste and harm. The huge cost to most who made early bets on a new general purpose technology. How many people died, were injured, or just plain lost their life savings by being first to deal with the dynamo in their workplaces - say in the first five or ten years it was available? We don’t know. How many factories suffered similar fates? How many businesses paid a bigger price than their gains - lost employees, slowed production, or went out of businesses entirely? Again, we haven’t really asked. But the lack of productivity gains proves the case: industry did not have major success through the technology before about 1910. Only a very few lucky firms would have achieved that success much earlier. That’s 30 years of near-guaranteed waste and failure - an entire adult’s working career!
We could ask similar questions about all general purpose technologies through history, from fire and the wheel through electricity, the internal combustion engine, telephony, the computer, the internet, and now, generative AI. The pattern in studies of technologies like these is clear: going early is risky, hard, and nobody really wins (or, more factually, lots and lots of firms and individuals lose) until we figure out the right ways to design and use them.
This is why - if you just take a cold, unemotional look at the facts - following is the best move. Unless you are simply compelled to try the latest new thing, you should do a little low-risk, high-potential-payoff experimentation with unalloyed generative AI, and to wait for the risk takers to figure things out. You’ll be fine.
The apps are coming, the apps are coming
The other reason why I’m confident you can wait to use generative AI is that we’re already seeing some green shoots when it comes to its utility for everyday people and organizations. The apps are coming.
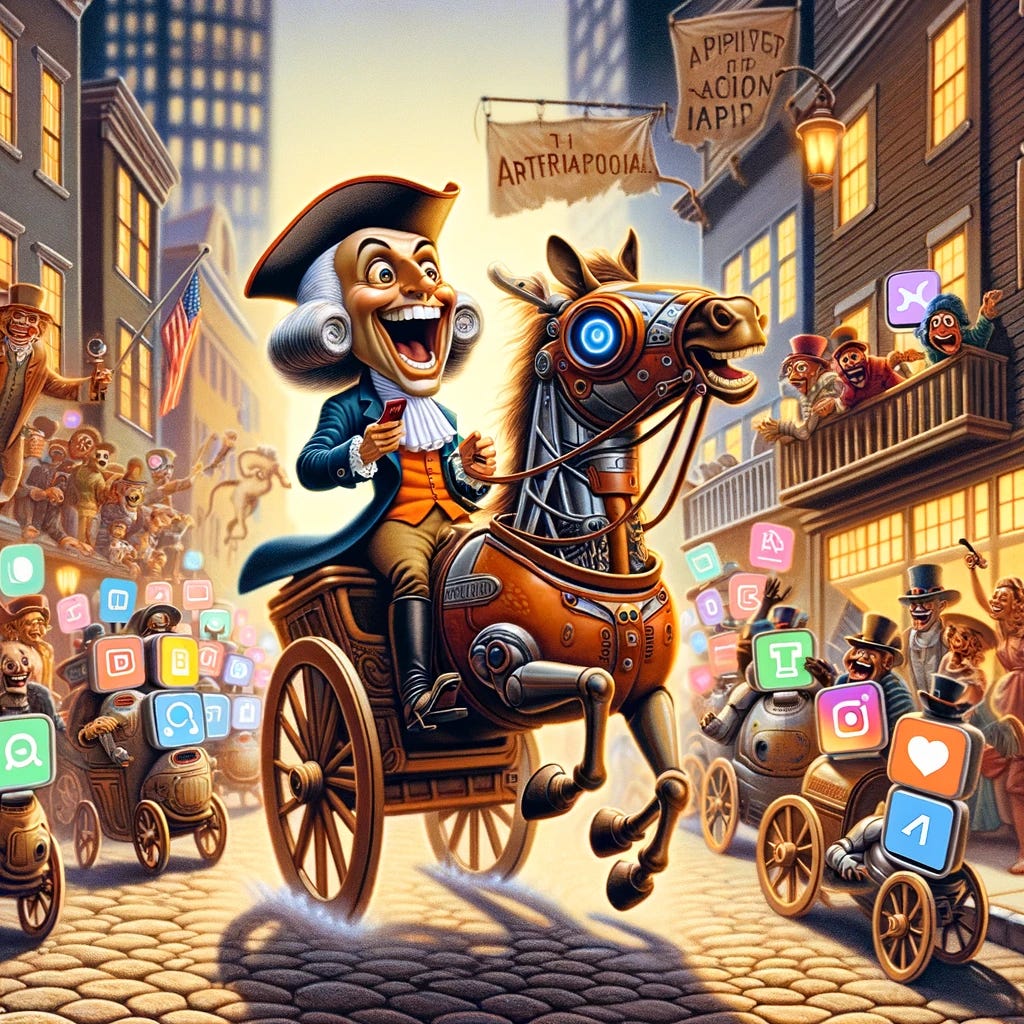
Think of generative AI as raw electrical power. An extremely powerful resource that can be used for lots of things. OpenAI’s big innovation was to package it up in a chat interface. That instantly made it more useful for billions of us. But that’s basically like getting electricity to your home. Fine, you have electricity, outlets, and electric motors on hand, but what can you do with them?
Most of us shouldn’t start messing around with motors, wire, and whatever we’d hook it all up to. We’d get electrocuted or maimed, or at best waste a ton of time. What most of us should do is wait for entrepreneurs to make reliable appliances. Lamps. Table saws. Dishwashers. Standup mixers. Heaters. Refrigerators. Water pumps. Then we can get to work buying those and putting them into our homes.
Appliances are specific, physical tools that make electricity and motors - both general purpose technologies - easy to use. When we get these for digital general purpose technologies like the internet or the smartphone, we call them applications, or apps for short. The first iPhone came with only a few apps - Jobs famously showed it as being “an iPod, a phone, and an internet communications device”. Then, eventually, they opened it up to developers who made thousands of apps, some of which made these devices far more useful.
Many firms are doing this right now for generative AI. You can bet your future on it. OpenAI and Google can’t do this alone. They need the world to work out how to make generative AI useful. So if it’s not your strong suit already - if you don’t already have a *very* clear line of sight to something innovative - your best bet is to stay a little connected, do some low-cost learning and experimentation on occasion, and wait until someone hands you something genuinely useful.
Microsoft’s new “Copilot” in their office suite provides a nice, easy example to think with.
Browse through that page. You’ll see: OpenAI’s LLM functionality has been woven into each of Microsoft’s office apps. Outlook for email, Word for documents, Excel for quantitative data, PowerPoint, and so on. In each, you get context-aware, personalizable tips, guidance, and automation that matches pretty well with your prior experience, goals, and skills. Their systems won’t just chat with you. With your permission, they’ll read your emails, prior writing, and maybe even consume your job description to give you help that’s tailored to your specific work. It can even “coach” you to improve over time - which has the potential to deal with the huge AI-threat to your skills. Using Copilot is kind of like going from a hand saw to a circular saw, powered by electricity. Slightly different game, same task. And the electricity/genAI is in the background, powering it all. You’re not dealing with it in it’s raw form. It’s appified.
Another example that’s a bit more out there comes from a startup called Lindy.ai. They’ve leapfrogged ahead of OpenAI to create a system that lets you spin up groups of AI agents that can independently cooperate with each other to handle complex projects. You create “Lindies” for each task syou care about - handling customer support, entering data, doing market research, taking notes, scheduling appointments - then you “onboard” them with a bit of text explaining their job, you connect them to your apps (like slack, google drive, calendar, docs, linkedin, zoom, github, and so on), and then they get to work solving problems for you.
OpenAI took a babystep in this direction a couple weeks back when they launched “GPTs” (agents you could program to be good at one thing - I made one to help with Harvard-style case writeups and one to help you write better prompts) but they’re not connectable to other apps, and can’t interact with each other. Small firms have huge incentives to unlock useful apps like Lindies, and we can count on them to do it, or die trying (many of them will).
This is what’s coming. For most anything you’ve used computers for before - from creating text and images, to sending and receiving messages, to browsing content, to video calling… whatever it is, it will soon be transformed into apps that are readily and reliably useable to you with very little retraining cost.
The lessons of history and your personal preference here are probably aligned: you shouldn’t go first, or early with unrefined general purpose technologies, generative AI included. Those who do have a good chance of hurting themselves pretty badly, and more commonly just waste a bunch of time and money.
Just take a deep breath, go about your business, and a new layer of helpful “intelligence” will be built into many of your day to day work tasks, probably sooner than you think.
ps: this is my fourth Substack post.
Many of you (over 250 in just under a month!) have been here from the beginning, and your positive feedback has inspired me to continue, though I will take a brief break over the holidays. Thank you!
I have a favor to ask. If you’ve found my writing valuable, please share it on social media, with colleagues, friends, and family. I’d greatly appreciate it - I only write about things that I think the world genuinely needs to hear right now, and I can only get these messages out there with your help. And by the way I’ll be sharing some exciting news with you all soon - before the rest of the world gets to hear it!
Matt, Thanks for the message not to get overwhelmed by AI - very easy for us older folks! I’m hanging on for dear life when I read about it all! Tell me, are the illustrations generated by AI?