The Specter of Skills Inequality
genAI allows us to get better output, faster - and learn faster, too. But only if we have resources: talent, networks, training, time. We don't want the future determined by those forces alone.
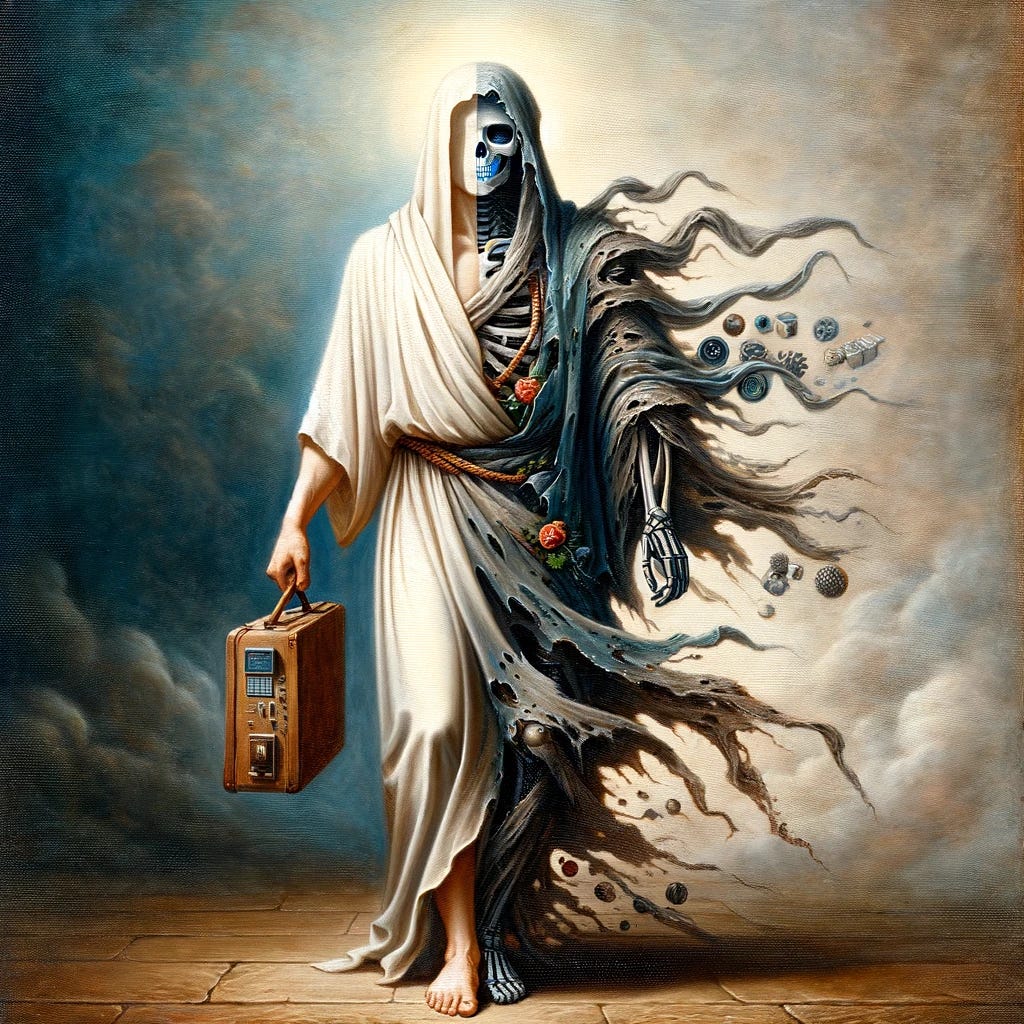
If you’re reading this, you’re probably fine.
You’ve been up on genAI for a while now. You followed ChatGPT back before it was cool - you probably remember when the “code interpreter” feature came out, and you might have even used ChatGPT 3. You quickly signed up for Google’s new Gemini Advanced - maybe even on the day it was announced in early February. And you likely know that just this Monday, Anthropic released Claude 3 Opus, a model that “outperforms” ChatGPT 4. You *might* even know what those airquotes were about: Anthropic benchmarked Claude 3 against ChatGPT 4 at release, meaning they didn’t compare against OpenAI’s latest, most improved model. In a highly competitive market, the sizzle sometimes overpowers the steak.
And if you know all this, you’ve also tried these systems out. You’ve gotten first drafts. Edits. You’ve written a funny, likely terrible poem or story (or twelve). You’ve created songs with Suno. You’ve generated code. You’ve asked for online research, and for synthesis of the results. You might even be that person who helps friends and colleagues get up to speed. You know enough to be helpful to a novice - getting them oriented, addressing questions and fears, teaching them the basics, and getting them started. And if you’re a loyal reader, you might have even taken the “try the impossible” challenge I issued a couple of months ago: from a cold start, use genAI to attempt a task you literally thought you couldn’t perform, no matter how hard you tried. One way or another, you’ve learned a lot - about what this technology can do, what it can’t, how it can mislead or confabulate, and how to handle that to get the results you need.
If you did all this and you swap your labor for money, you likely do work that is significantly “exposed” to generative AI - in other words you can use genAI to get better, faster results on a healthy chunk of your work tasks. Given that genAI can’t interact with the physical world, these tasks are overwhelmingly cognitive - produce writing, analyze data, write code, create or analyze images, reconsider or craft concepts. Imagine anything you could do remotely and you’ve got your arms around this kind of task.
But I can also predict a few other things about you. The first follows pretty directly from the prior paragraph: you probably earn(ed) a pretty good living. The kinds of tasks above - the kinds that genAI can help with - are on the cognitive/social side of the range of human skills. Those are the skills that our society pays for, and it pays a lot more for them in many cases. Most sensorimotor skills just don’t get the same pay. Yes, the middle class that handled more routine cognitive work has been hollowed out over the last forty years or so, but those who are left are dealing with real, complex problems. If you’re in finance, HR, marketing, or some other internal function, your job might be a bit too routine for your taste, but it requires judgement, emotional intelligence, and more. So, fine, you’re doing okay financially, and you’re in what many labor economists would call a “high skill” job (I strongly object, but that’s another story).
Next: you’ve got discretionary time. Nobody’s studied this yet, but “getting” the relatively unrefined genAI we’ve been given requires a lot of experimentation, and I think a lot of that is lonely failure. Trying things out, getting confused and frustrated, ending up without an answer and giving up, finding out a long chain of prompting isn’t really worth it for the results you got, searching online for tips and finding underinformed hype, keeping up on the news of it all, barely pausing to wonder who else is doing this, let alone connect with them… all in exchange for a few eurekas and a small pile of insights that allow you to be reasonably efficient with it today. It’s not obvious you’ve come out ahead on pure productivity terms. Most haven’t. In fact late last year I used this logic to argue that most of us should probably cool our jets on genAI. It’s a time sink, but let’s face it, you’ve got the time to burn.
Relatedly: you’re a bit of a deviant. Even now, genAI is like electricity and a large electric motor in a previously nonelectrified home: you can get a lot of value out of it, but you’ve got to be willing to do something that you’ve never seen before, and you can’t guarantee it’ll be effective, appropriate, or safe. In the midst of your efforts to “get” your new electric motor there would probably be a moment (or twelve) where if someone walked in on you, they’d see a Rube-Goldberg style contraption made of belts, pulleys, boards, braces, and maybe a feather duster. You would likely end up with a few literal scars or burns to show for your efforts, and your family would at best tolerate the disruptive panjandrum in your living room. All en-route to an automated dusting device. Obviously using genAI is a lot more straightforward than all this, but it’s metaphorically the same: you have to be willing to bend the rules and strain propriety to learn your way to productivity. This mixture of deviance and progress goes hand in glove with “shadow learning”: the kind of skill development I uncovered in my research on robotic surgery.
To knock off a few more: you’ve probably got solid internet access, are based in North America or Europe, and can think and work well in English. The internet access bit might seem obvious, but that’s because you’ve got it. Less than 1/3 of the world’s population enjoys the same privilege. Then there’s the geography bit: OpenAI, Google, and Anthropic have made their systems available first in the United States and second in Canada and Europe. Are you a world-renowned economist who wants to use Claude 3 Opus for research on release day, but live in Canada? Sorry. Some of the reason is doubtless geopolitical. But some of it is linguistic. These foundation models are trained on the internet, but really if you look under the hood they’re trained on masses of text in English. All serious firms are working their way out from there, but their internal cultures, training data, marketing, demonstrations, support capabilities and so on are all rooted in English.
So, to sum up: you’re probably do work that’s significantly “exposed” to genAI, earn(ed) a good living, have discretionary time, bend the rules and defy norms more than most, have solid internet access, are based in North America, and speak English.
Most humans don’t.
And that means - when it comes to the skills needed for the wild new world of work we’re inhabiting - you’re probably going to win, and they’re probably going to lose.
This is the specter of skills inequality.
The available science suggests this is fractal - occurring on the individual, group, organization, industry, culture, and national levels. As an individual you have the resources needed to engage with genAI, you have a much better shot at building skill with it than someone who doesn’t. And if it enhances your productivity, you’re going to get access to that next valuable opportunity more, better, faster than they can. Sometimes, of course, you’ll lose. Fall on your face. Waste your time. Hurt someone. So it’s a bit tricky, but—on average—you’ll seize opportunity.
But things get trickier from there. What makes for skills inequality at the individual level might have different effects at other levels. I found this in my study of robotic surgical training, and called it a “Matthew effect for skill”: the better you did with the technology, the more practice you got, and that came at your peers’ expense. They build less skill. So that group - and, by proxy, that occupation - saw severe capability limitations over time. You might see something comparable if everyone (or even most everyone) tried to use genAI all at once. Semi-focused, variable and spiky productivity, and the performance at the group level could seem simply meh.
Skills inequality doesn’t necessarily mean trouble for groups and organizations. If a few people or groups race ahead by design and people can plan around that, the collective could learn from their experiments, optimize them, and then race ahead in unison. In a sense this is what formal R&D departments do in large organizations. Learning and development professionals and functions can play a critical role in enabling this kind of thing. Social systems are fiendishly complex, so there will be other, surprising ways that skills inequality is a tide that raises all boats.
Sociology trumps agency
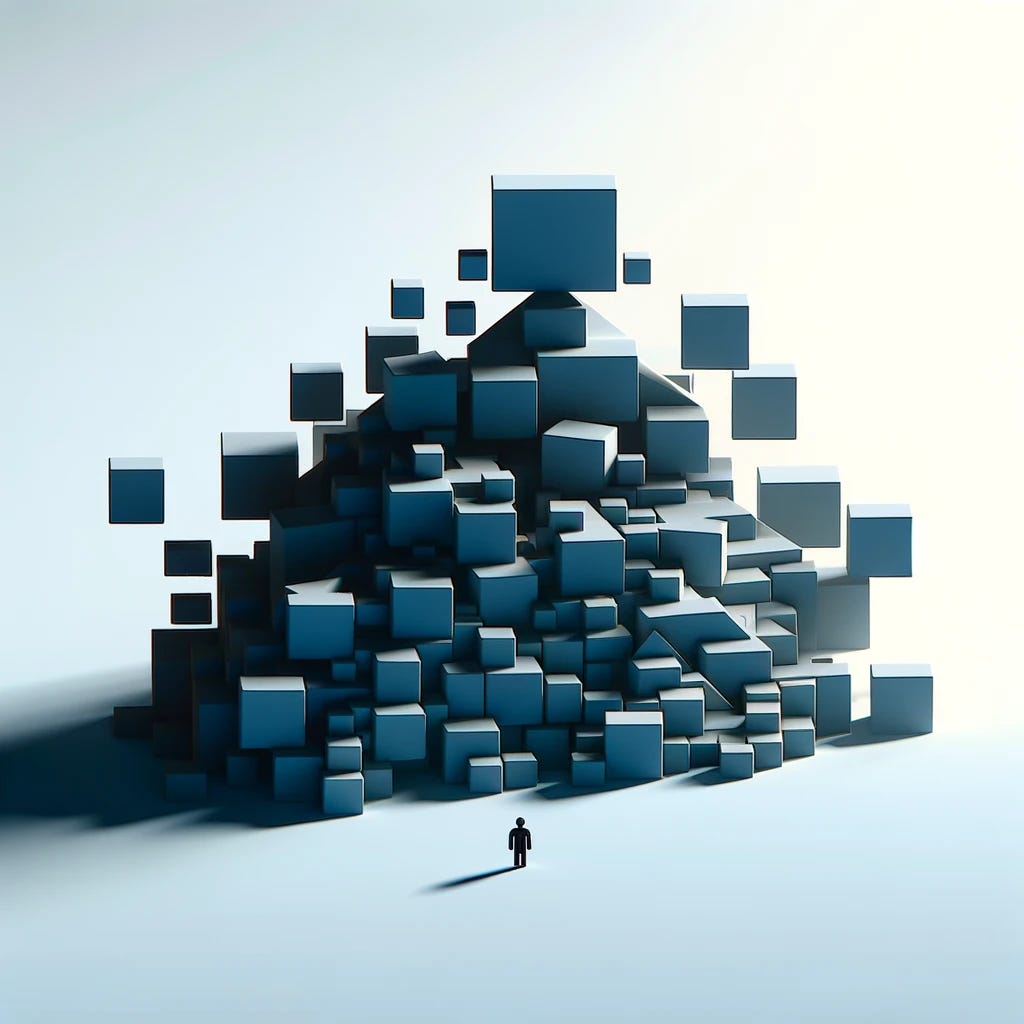
But the sociologist in me doesn’t operate on hope, positive exceptions, or possibilities. It sees social forces at work. Situations, practices, tools, institutions, and cultures that make certain outcomes more likely for anyone who gets involved. Deprive someone of resources like money and, on average, they start to behave and think like a poor person. Put someone in a social network with a certain set of political beliefs and, on average, they’ll adopt them. Give us technology that allows us to get rapid productivity gains if we reduce novice involvement in the work? On average, we’ll do it. From this point of view, the “safe” prediction from all the research I’m aware of—mine and others’—is that we will continue to seek immediate productivity from genAI and other intelligent technologies. As individuals succeed with less help, the collaborative bonds between those who can and can’t will fray. We will degrade healthy challenge, complexity, and connection - the necessary components of skill development that comprise the skill code at the core of my upcoming book. The rare few who find shadow learning practices or who are lucky enough to avoid these traps will race ahead with far more skill than the rest of us.
We worry about income and wealth inequality these days. Serious problems. But right now, we are also racing down a road toward skill inequality, and the sociologist in me doesn’t see any forces arising to change that on a mass scale.
We do not want to live in that future. First off, individuals who hit a wall on skill development will hit a wall on their careers, their income, and their quality of life. For most surgeons, this might mean getting blocked from top-tier hospitals, limits to income, and the stress of being behind the times—while a few race ahead to far more prestige, more miraculous patient “saves,” and dramatically higher income. But skill inequality goes way deeper and darker than it will in high-status, highly paid professions. After spending three years interviewing hundreds of warehouse workers with my team, I can tell you firsthand that the aphorism attributed to William Gibson (“the future is already here—it’s just not evenly distributed”) is just as applicable here as in robotic surgery: this darker future is already here, and it’s heavily concentrated in low-wage, entry-level, repetitive work. These folks are often temporary workers. No benefits, no job security. Barely any training, practically zero mentorship. And warehousing corporations invest massive resources to aggressively deskill the jobs these folks occupy. Industrial engineers walk the floors, doing detailed time-motion studies to figure out how they could improve productivity. For a warehouse? That’s how many items the building can process in a day, times a “defect” rate—or the percentage of units out of a hundred that don’t get damaged, badly labeled, stolen, and so on. And what’s the best way to increase throughput times quality? Reduce “skilled touches.” Those industrial engineers know that every time a human has to deal with an item, there’s a chance for an error. And that the more skill it takes to handle that item, the greater the chance of the error is. So they are continually redesigning the work to extract skill requirements from the job and quantify the output—not just reduce the number of times a human has to interact with the product. It’s only one more step to the stark conclusion: the longer that a worker stays in jobs like this, the less skill they can expect to have over time. Their skill degrades.
That’s degrading, too. A lot of folks, according to one worker, get to a place where they
“are content with just doing the grunt work. I mean because a lot of these pick and pack places—it’s like high school, do you know what I mean? You’d rather just stay in your lane in the palletizing and don’t even get involved with the people up there [in other areas].”
So long, healthy challenge and complexity. So long to healthy connection, too. Said another one:
“Honestly, I can’t [tell you about what my coworkers are like] because I don’t talk to anybody there. We’re trying to go so fast to make our numbers.”
And this last worker tells us the nasty conclusion—the longer you stay in a job like that, the less likely you are to look for something better:
“there’s a lot of warehouse jobs that you see people after being there for a while, and I just can tell they’re drained, tired all the time, and just fed up of it but [they] don’t really know anything else.”
Here and in many comparable places throughout the economy, we really are destroying human potential on a mass scale in the name of techno-productivity. The rare few shadow learners that my team and I found in warehouses all around the US prove that we can build rich, valuable skill just about anywhere. Deskilling, degrading work is always optional. On top of that, these workers’ innovations show us that favoring skills over pure productivity isn’t an act of charity or empathy alone. Insisting on skill development and productivity offers huge competitive business advantages, too—and opportunity costs if we stick with the skill-sacrificing status quo.
A world where the skill code is healthy and vibrant will not write itself. Without a focused, massive investment, we’re going to get more of the skills future I saw unfolding for most workers in warehouses. To pull this off—to stop the dark sociological skills prediction from coming true—we must build a new, global, AI-enabled infrastructure to strengthen these foundations of skill instead of undermining them. On that score, we need to take the very technologies we’re concerned about—robotics, AI, cameras, the internet, mobile devices, and now large language models like ChatGPT—and use them to build the infrastructure for skill we need in the twenty-first century. And those with power in organizations need to weave this new infrastructure into everyday operations so they’re more powerful than they would be otherwise. This is a future that brings humanity and technology into an even tighter dance where challenge, complexity, and connection thrive.
The (Healthy) Future of Skill is Chimeric
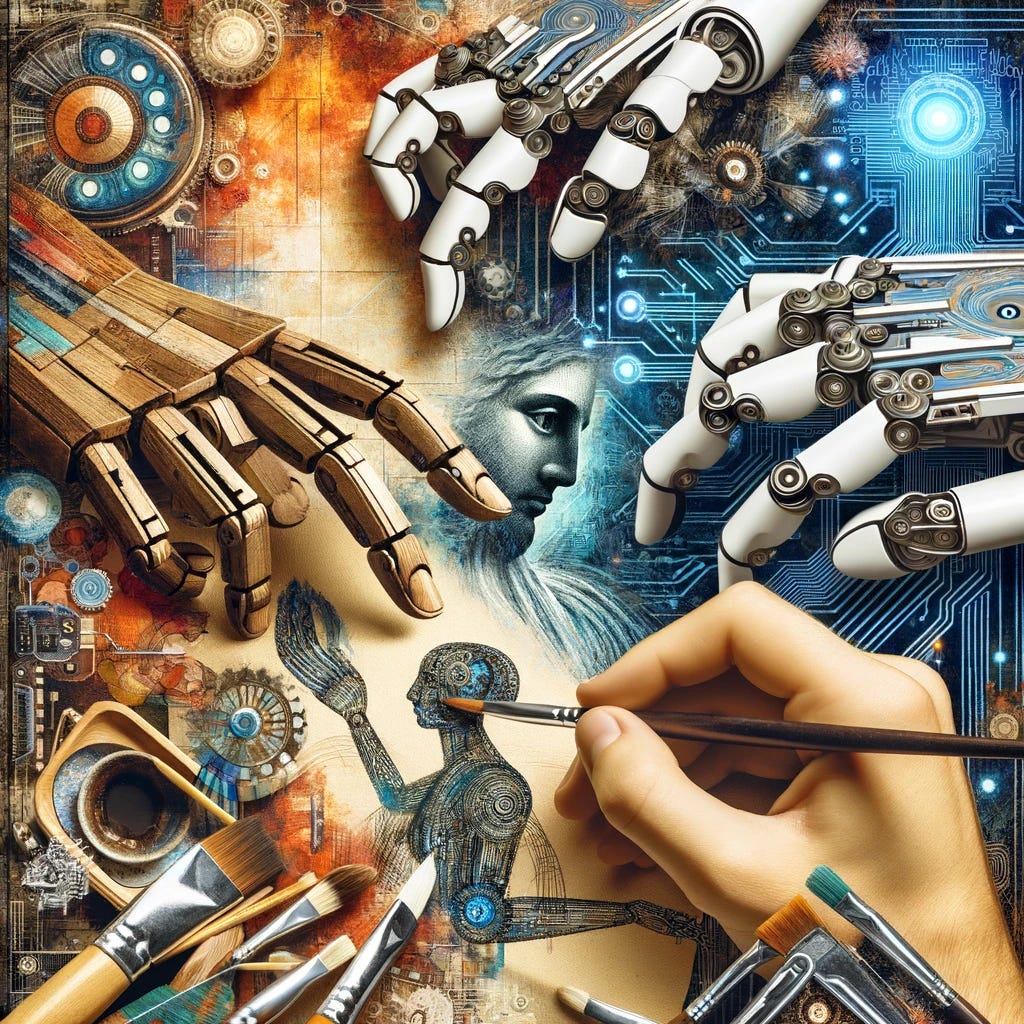
In this new world, we would have a network of human experts, novices, and AI, focused on building human and AI capability right in the middle of work. This could begin with AI-assisted matches between experts and novices who happen not to work in the same physical space or organization. Firms are already doing this in coarse ways, and they’re starting to blend genAI into their solutions. But a healthy future for skill will mean a comprehensive new fabric for the expert-novice connection—where simply by engaging with it, both humans and AI learn faster than they could on their own, enhancing human relationships and our sense of fulfillment along the way. A lot of the tools to enable this are already on the table: human-computer interaction researchers like Kurt Vanlehn and Michelene Chi at the University of Arizona have shown us interactive, automated systems that provide powerful assistance and even collaborative glue as learners seek skill. Sometimes these help us build skill more than a human tutor would—a bounded but real solution to Bloom’s “two sigma” problem (basically that 1:1 tutoring is far better for student learning than 1:many). Generative AI offers significant opportunities to enrich and deepen this human-technology partnership, and our collective skill along with it.
We’ve started calling systems like these chimera. This was originally the name for an ancient Greek creature with a lion’s head, a goat’s body, and a serpent for a tail. A blend of different entities. A system is chimeric when it is neither human nor technological—one that allows us to take full advantage of both in ways that do better than either humans or AI could do alone. An early, clean example of this is in the world of chess. For centuries, humans were best at the game, competing and examining the game with fearsome intensity to advance the state of play. Then, in 1985, computer scientists at Carnegie Mellon started to develop a chess program they called “Chip Test.” But you probably know it by the name that IBM gave it after they took on the project in 1989: Deep Blue. In 1997, it won an exhibition match against Garry Kasparov, the great chess grandmaster. That was it, apparently: computers were best at chess. But it didn’t last. Soon the best chess players were chimera—a human partnering with an AI won against a human alone or an AI alone. The AI could propose a complex tree of moves that had a high probability of success, given the lay of the board. But the human was best at intuiting play styles and taking considered risks. Ultimately AI rose to the top again, but chess isn’t a complex problem compared to real life. When the board, the rules, and the number of players shift in unpredictable ways, chimera will have real staying power.
Some companies are using chimeric systems to help people become more effective in their jobs—like maintaining gas turbines, laying out computer chip designs, operating on cancerous tumors, and even harvesting crops. When designed carefully and deployed well, chimeric solutions scaffold the human to more productivity than either human or technology could achieve alone: subtle, early preventative maintenance that keeps a turbine spinning for less cost, ingenious chip layouts that save power, being sure you’ve gotten all the cancer in an operation, and doing far less damage to a crop as you navigate a combine with hyperhuman precision. But as my research shows, today’s chimera almost never develop that human’s skill in the process. They don’t promote healthy challenge, foster healthy complexity, or facilitate healthy connections between humans. In the short run, using intelligent technologies gives us great productivity; chimeras can sort tomatoes or trade securities like a fiend. But then the humans in that loop forget how to do these things and there is no chimeric skill-building system in place to redirect, utilize, and enhance that person’s intelligence and capability.
We don’t have a digital apprenticeship to replace the analog one we’re losing. To save human ability in an age of intelligent machines, we’ve got to build one. Starting right now.
You did get the absolute soul read on me and my aspects, although I think I at least have some minor significant thing to show for it already, in that "I" can now do quick low level python scripting which I couldn't do before, and is a huge time save.
I've AI Narrated this post with ElevenLabs, if that's OK.
https://askwhocastsai.substack.com/p/the-specter-of-skills-inequality?sd=pf